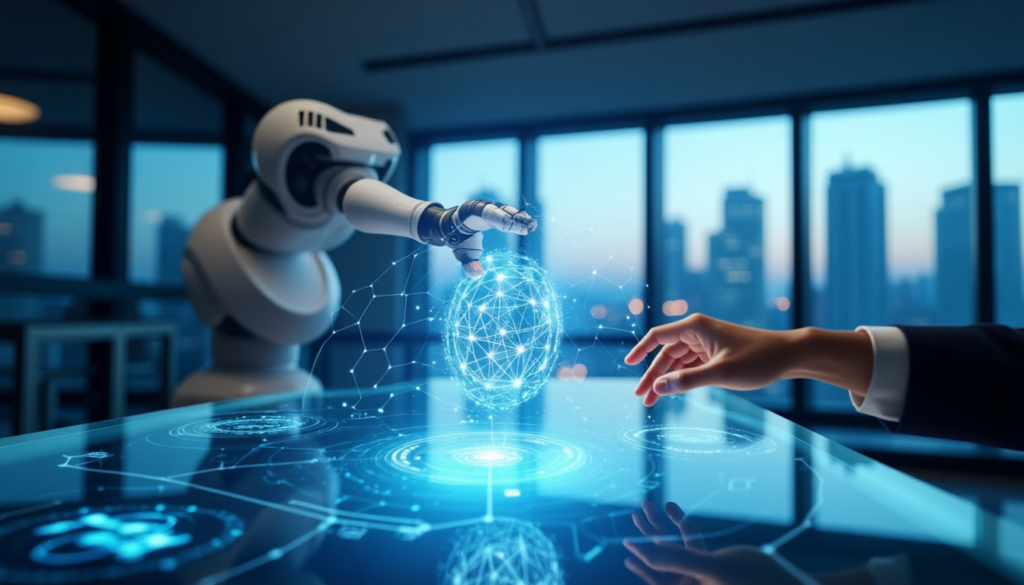
Thousands of businesses struggle daily to keep up with fast-moving technology changes. Their competitors already exploit AI business solutions to gain the upper hand. AI has become crucial to propel development in today’s business world.
The thought of implementing AI in business might seem daunting. We created this detailed guide to direct you through the AI world. Our practical steps will help you utilize AI effectively – from checking your organization’s readiness to tracking ROI.
The guide simplifies AI adoption into clear steps. You’ll learn to dodge common mistakes while getting the most from business AI. We cover infrastructure needs and staff preparation to give you the tools for successful AI implementation.
Understanding AI’s Business Transformation Potential
Businesses are adopting AI at a remarkable pace, with 72% of organizations now implementing AI solutions. AI has evolved from being just another business tool to becoming essential for staying competitive.
Current state of AI in business
The digital world of business AI keeps growing faster. Recent findings reveal that 65% of organizations are regularly using generative AI in at least one business function – almost twice as many compared to ten months ago. The numbers tell an interesting story: half of all companies now use AI in two or more business functions. This shows how versatile AI has become in businesses of all sizes.
Key benefits and opportunities
Companies are seeing remarkable results from AI implementation in several areas:
- Enhanced Efficiency: AI takes care of repetitive work so employees can tackle strategic tasks
- Data-Driven Decisions: Immediate analysis of big data sets leads to deeper business understanding
- Customer Experience: AI-driven personalization creates happier, more loyal customers
- Innovation Acceleration: Teams can focus on research and development when AI handles routine tasks
Common misconceptions and challenges
Many people think AI will replace human workers completely. The reality shows that AI works best as a tool to improve human capabilities, not replace them.
Businesses face several major hurdles when implementing AI:
- Data Quality Issues: Quality and quantity of data often fall short of AI requirements
- Infrastructure Gaps: Most businesses still grapple with outdated systems that make AI integration challenging
- Expertise Shortage: Finding skilled professionals for AI implementation remains difficult
- Risk Management: 44% of organizations have experienced negative consequences from AI use, with inaccuracy leading the list of problems
The numbers raise concerns: only 18% of organizations have an enterprise-wide council for responsible AI governance. This oversight gap becomes more critical as more industries adopt AI.
Yet signs of progress in AI implementation look promising. Companies take a more strategic approach, and 67% plan to increase their AI investments over the next three years. This mix of growth commitment, careful risk management, and clear goals helps businesses discover the full potential of AI.
Developing Your AI Implementation Strategy
A well-laid-out strategy leads to successful AI business implementation. Organizations that achieve the best results take a step-by-step approach to AI adoption. They focus on basic elements before scaling up.
Assessing organizational readiness
AI success doesn’t just depend on technology. Our research shows that strategic, organizational, and cultural factors matter just as much. You need a complete readiness assessment to get into your current capabilities and find areas that need improvement.
Here are five key stages of AI readiness we assess:
- Exploring: Focus on building AI strategy and experience
- Planning: Concentrate on formalizing business strategy
- Implementing: Develop leadership support and scale expertise
- Scaling: Create an innovation culture
- Realizing: Encourage continuous breakthroughs within teams
Setting clear objectives and KPIs
Companies that use AI to create new KPIs succeed more often. These organizations are three times more likely to see greater financial benefits than others. Your objectives should measure AI success through both direct and indirect metrics.
Smart KPIs should be:
- Descriptive: Synthesizing historical and current data
- Predictive: Anticipating future performance
- Prescriptive: Making AI-recommended suggestions for improvement
Creating an AI roadmap
Digital transformation needs a company-wide approach. Organizations in the planning stage should prioritize business strategy. This helps arrange AI use cases with business objectives.
Your AI roadmap should progress in phases, with each stage building on previous work. Research shows that 66% of respondents view AI as critical to success. However, only 38% believe their use of AI separates them from competitors. Here’s how to close this gap:
- Start with experimentation
- Deploy for productivity
- Transform experiences
- Build new capabilities
Only 40% of organizations have a company-wide strategy. Your roadmap should have clear governance principles and priorities for AI implementation to avoid this issue. Organizations that communicate a clear vision are 1.5 times more likely to achieve desired outcomes compared to others.
Building the Right Infrastructure
A strong infrastructure is vital to implement artificial intelligence in business. The foundation of successful AI adoption depends on three key areas: technical architecture, data management, and security frameworks.
Technical requirements and considerations
Successful AI programs need a complete technical foundation. Organizations must have both hardware and software capabilities to handle complex AI operations well. These essential components will help:
- High-performance computing resources
- Expandable cloud infrastructure
- Integration capabilities with existing systems
- Strong data pipeline architecture
- Strong storage solutions
Data management and quality
Data quality serves as the life-blood of effective AI implementation. Studies show that poor data quality is the main obstacle to deploying AI projects. Organizations that achieve the best results follow these significant steps:
- Establish clear data labeling processes
- Implement strong data validation protocols
- Create unified data access points
- Maintain consistent data formats
- Regular data quality assessments
Our analysis shows that AI programs must be trained to represent reality accurately, and successful models require precise data. Bad data can cause more problems than no data at all. Quality data should be your priority from day one.
Security and compliance frameworks
Security must be built into AI business implementation from the start. 89% of surveyed companies lack proper tools to secure their AI systems. Organizations need complete security measures that match industry standards to address this gap.
Successful organizations implement AI security through multiple layers. They protect sensitive data, ensure model integrity, and maintain regulatory compliance. AI-driven security tools help prevent data breaches and ensure compliance with regulations like GDPR and PCI-DSS.
The core team focuses on three key areas to manage compliance:
- Data Protection: Implementing encryption and access controls
- Model Governance: Establishing frameworks for security testing and ethical compliance
- Continuous Monitoring: Regular auditing and performance assessment
Organizations with strong governance frameworks handle AI-related risks better. This becomes especially important as 35% of companies report using AI in their business operations, with an additional 42% learning AI implementation.
Creating an AI-Ready Workforce
The business world is changing faster than ever, and building an AI-ready workforce has become a top priority. Our research shows that 38% of workers will need fundamental retraining or replacement within three years to fill workforce skills gaps.
Skills assessment and gap analysis
A full picture of skills helps businesses implement AI successfully. Data shows that 43% of businesses experienced skills gaps in 2020, which substantially affected their performance and employee satisfaction. The best way to start is with a detailed skills inventory that assesses:
- Technical capabilities
- Data literacy levels
- Problem-solving abilities
- Adaptability quotient
- Collaboration skills
Training and development programs
Our data shows employees want to learn, with 68% ready to retrain whatever the circumstances. Training programs need multiple components to work well. After setting up well-laid-out development programs, 90% of technologists used learning platforms.
AI business training works best when you:
- Develop customized learning paths
- Implement AI-powered learning platforms
- Create hands-on practice opportunities
- Establish mentorship programs
- Regular progress assessment
Change management strategies
AI adoption needs strong change management to succeed. Research shows that resistance can be a vital source of insight with a strategic approach. We create “AI playgrounds” where employees can try business artificial intelligence without affecting operations.
Organizations that set up Centers of Excellence (CoE) see great results. These centers help centralize expertise and codify best practices. Research shows that 60% of IT decision-makers think AI skills shortages threaten their business.
Clear communication about AI’s role helps drive adoption. Organizations with a clear vision implement AI more successfully. 70% of workers need better AI skills, so creating supportive learning environments matters.
Success in AI business implementation comes from mixing technical training with cultural change. Companies should focus on upskilling current employees and creating paths for new talent, as 55% of employees say they need more training to do their jobs better.
Implementing AI Solutions Step-by-Step
Success with AI business solutions comes from starting small and growing smart. Research shows that 80% of industrial AI projects can fail to generate tangible value. The right implementation is vital for success.
Pilot project selection
The right pilot project lays the foundation for AI success. Data from successful organizations reveals a systematic approach to pilot selection. Here’s what we look for:
- Quick-win potential
- Industry-specific relevance
- Clear value creation
- Manageable scope
- Available expertise
Starting with existing systems works best. Research confirms that integrating AI with current software often yields faster results. We help teams find ways AI can improve existing processes before adding new systems.
Scaling successful implementations
Teams need guidance after their pilot succeeds. Our research shows that organizations using systematic approaches successfully scale more than twice as many AI use cases compared to teams without structured methods.
These elements drive successful scaling:
- Data Products: Feature stores cut time-to-market
- Code Assets: Reusable packages support long-term sustainability
- Standards: Resilient frameworks help evaluation and deployment
- MLOps: Machine learning operations enable efficient scaling
Measuring and optimizing results
AI business implementation needs solid measurement strategies. Our data reveals that most AI/machine learning projects focus solely on technical metrics, which often leads to project failure.
We track both technical and business metrics. Key areas include:
- Return on Investment: Financial benefits versus expenses
- Adoption Rate: Usage numbers across departments
- Customer Experience: Satisfaction scores and participation
- Time-to-Value: Implementation speed
- Model Accuracy: Technical performance
Regular monitoring makes a difference because AI models will eventually degrade or become obsolete due to changes in data or business requirements. We run assessment cycles to maintain peak performance and line up with business goals.
Experience shows that organizations achieve the best results when they monitor and optimize rigorously. They conduct regular workshops and cross-functional team meetings to keep AI solutions working and aligned with business goals.
Managing AI Risks and Governance
AI business implementation risks have become more important as organizations face new challenges in AI governance. Our research shows that 84% of workers using generative AI have accidentally exposed company data in the past three months. This highlights why we need resilient governance frameworks.
Ethical considerations
Business AI implementation needs careful attention to ethical principles. Our data shows that only 18% of organizations currently have an enterprise-wide council for responsible AI governance. We want to close this gap by focusing on these key principles:
- Transparency in AI decision-making
- Fairness in algorithm design
- Accountability in AI systems
- Protection of privacy rights
- Commitment to ethical AI development
Regulatory compliance
Business AI regulations are changing faster than ever. Major changes are happening, with nearly a dozen US states enacting AI-related legislation. Organizations must get ready for complex regulations that have:
- Data privacy regulations (GDPR, CCPA)
- Industry-specific compliance requirements
- Emerging AI-specific legislation
- International regulatory frameworks
Our data shows that many organizations don’t want to adopt AI applications because they worry about privacy, security threats, and copyright infringement. Organizations should establish clear AI principles and guidelines that line up with their ethical values.
Risk mitigation strategies
We take a practical approach to business AI risk mitigation. Organizations need to develop capabilities to detect, identify, and prevent misleading content from spreading.
Large AI providers now take responsibility for intellectual property infringement claims. But we still recommend strong internal controls. Our research shows successful risk mitigation needs:
- Regular AI system audits
- Complete documentation processes
- Clear accountability frameworks
- Continuous monitoring protocols
- Incident response planning
Watermarking works well as a transparency tool that helps users tell AI and human-created outputs apart. On top of that, organizations need strong cross-checking systems because no automated systems can reliably identify whether any output is created by AI.
Strong governance needs internal guardrails and training programs. Our data shows that organizations that communicate clear governance and risk management practices handle AI-related challenges better. Businesses should focus on technical and organizational aspects of risk management. This includes regular updates to security protocols and compliance frameworks.
Our experience with business AI implementations taught us that good risk management balances new ideas and control. Clear communication channels and regular updates about AI governance practices help build trust. This ensures AI initiatives succeed in the long run.
Fostering Innovation with AI
AI powers businesses by sparking breakthroughs and solving problems creatively. Our research shows that 75% of teams using AI report discovering unique questions that changed their organization’s direction.
Creating an innovation culture
A successful AI implementation needs more than just technology – it needs a fundamental change in culture. Organizations succeed when they treat AI as a partner and collaborator instead of just a tool. These essential elements build this culture:
- Clear leadership vision and support
- Cross-departmental collaboration
- Knowledge sharing platforms
- Regular innovation forums
- Continuous learning opportunities
The results become interesting at the time AI combines with human-centric skills to improve our understanding of complex business environments. Companies achieve better results when they treat AI as a knowledge-work partner in product design and process efficiency.
Encouraging experimentation
“AI playgrounds” give teams safe spaces to explore and test new ideas. Our data shows that companies that provide resources and time for AI experimentation are 94% more likely to see employees trying AI for work-related tasks.
These key steps help maximize experimental value:
- Start with low-risk projects
- Document learnings and insights
- Share successful use cases
- Scale proven solutions
- Celebrate innovation wins
Teams become better problem solvers with AI tools that help them ask smarter questions and become breakthrough innovators. Organizations now approach challenges and develop solutions differently.
Managing resistance to change
Employee fears about job security often cause resistance to AI. Most AI initiatives fail due to employee-perceived threats rather than technical challenges. Clear communication helps employees understand how AI improves their capabilities rather than replacing them.
Employee involvement in the AI experience creates success. Our research shows that workers who feel trusted by their employers are significantly more likely to participate with AI tools. Trust transforms resistance into advocacy naturally.
“Catalytic questions” work well by pushing leaders and teams out of their comfort zones while promoting innovative thinking and action. Teams start seeing AI as a chance rather than a threat.
Successful organizations create environments where questions that spark change can emerge naturally. Regular knowledge-sharing sessions and collaborative problem-solving make this approach more effective.
Measuring ROI and Business Impact
AI business initiatives need a smart approach that goes beyond basic ROI calculations. Research shows organizations using AI-powered KPIs are five times more likely to effectively arrange incentive structures with objectives compared to those using legacy KPIs.
Key performance metrics
Success in AI business implementation needs both direct and indirect metrics. ROI in AI should be assessed across multiple dimensions. These include creativity, time saved, user satisfaction, and accuracy in generating outputs.
These KPI categories are the foundations of effective measurement:
- Smart Descriptive KPIs: Blend historical and current performance data
- Smart Predictive KPIs: Anticipate future outcomes and highlight risks
- Smart Prescriptive KPIs: Provide AI-recommended corrective actions
- Operational Metrics: Measure business process improvements
- Business Value Metrics: Calculate financial effect
Companies using AI to boost KPIs see remarkable results. To cite an instance, an AI solution that reduces human design time by 50% becomes a clear measure of ROI.
Impact assessment frameworks
Our work with AI business implementations has helped us create detailed assessment frameworks. Organizations need structured approaches to assess AI effects across multiple dimensions. Research indicates companies using AI to prioritize KPIs are 4.3 times more likely to see improved arrangement between functions.
Impact assessments focus on three core areas:
- Technical Performance: Measuring model accuracy and efficiency
- Business Operations: Evaluating process improvements and cost savings
- Strategic Value: Assessing long-term competitive advantages
Successful organizations use AI to find interdependencies among indicators. This creates “KPI ensembles” that bundle distinct metrics for connected business activities.
Continuous improvement strategies
Continuous improvement is vital to maintain ROI in business AI. A culture of continuous improvement makes successful adoption and integration of new technologies easier.
These proven strategies lead to optimal results:
- Regular Assessment Cycles: Monitor and evaluate AI performance consistently
- Predictive Maintenance: Use AI to anticipate and prevent issues
- Process Optimization: Continuously refine AI models based on outcomes
- Feedback Integration: Incorporate user insights and operational data
Organizations with strong governance frameworks handle AI-related risks better and maintain continuous improvement. Research shows companies using AI-enabled KPIs are more likely to improve arrangement between functions.
AI reshapes performance measurement fundamentally. Forward-looking organizations benefit from AI-generated KPIs that are more intelligent, adaptive, and predictive than legacy indicators. This has created “smart KPIs” that provide predictive insights and situational awareness. These help organizations improve performance and encourage better coordination among corporate functions.
ROI measurement needs a balanced approach. Tracking quantifiable metrics matters, but intangible benefits are equally important. Data shows organizations using systematic approaches successfully scale more than twice as many AI use cases compared to those without structured methods.
Successful organizations increasingly use AI to boost KPI information sharing and collaboration. Research indicates companies that use AI to share KPIs are five times more likely to improve arrangement between functions and are three times more inclined to be agile and responsive compared with organizations that don’t.
Conclusion
AI adoption has evolved from a competitive edge to a necessity for business survival and growth. Organizations that take a strategic, well-planned approach to AI integration achieve substantially better results than those who rush into adoption without proper preparation.
Three key areas demand careful attention for AI success: strong infrastructure development, complete workforce training, and solid governance frameworks. Companies that excel in these areas experience faster implementation cycles and stronger ROI. They create environments where AI and human capabilities work together effectively and drive state-of-the-art solutions for eco-friendly growth.
Building strong foundations should be the priority for organizations starting their AI experience. Quality data, skilled teams, and clear governance policies form the cornerstones. AI implementation isn’t a race to the finish line but a strategic transformation that needs patience, planning, and continuous optimization. Companies that adopt this mindset while focusing on measurable outcomes can tap into AI’s full potential.
FAQs
Q1. How can AI be leveraged to drive business growth? AI can drive business growth by automating routine tasks, enhancing data analysis, improving customer experiences, and fostering innovation. It can help create business content, develop marketing strategies, and provide valuable insights for decision-making.
Q2. What are the key components of a successful AI implementation strategy? A successful AI implementation strategy includes a clear vision, value realization plan, risk assessment, and adoption strategy. It’s crucial to focus on building strong foundations like quality data, skilled teams, and clear governance policies.
Q3. How does AI contribute to business innovation? AI contributes to innovation by enabling businesses to ask smarter questions, discover unique insights, and solve complex problems. It can enhance product design, improve process efficiency, and help teams approach challenges from new perspectives.
Q4. What are the main challenges in implementing AI in business? Common challenges include data quality issues, infrastructure gaps, skills shortages, and resistance to change. Additionally, managing AI risks and ensuring ethical use are significant concerns for many organizations.
Q5. How can businesses measure the ROI of AI implementations? Businesses can measure AI ROI through a combination of direct and indirect metrics. These include operational improvements, time saved, user satisfaction, accuracy of outputs, and financial impact. It’s important to use AI-enhanced KPIs that provide predictive insights and situational awareness.