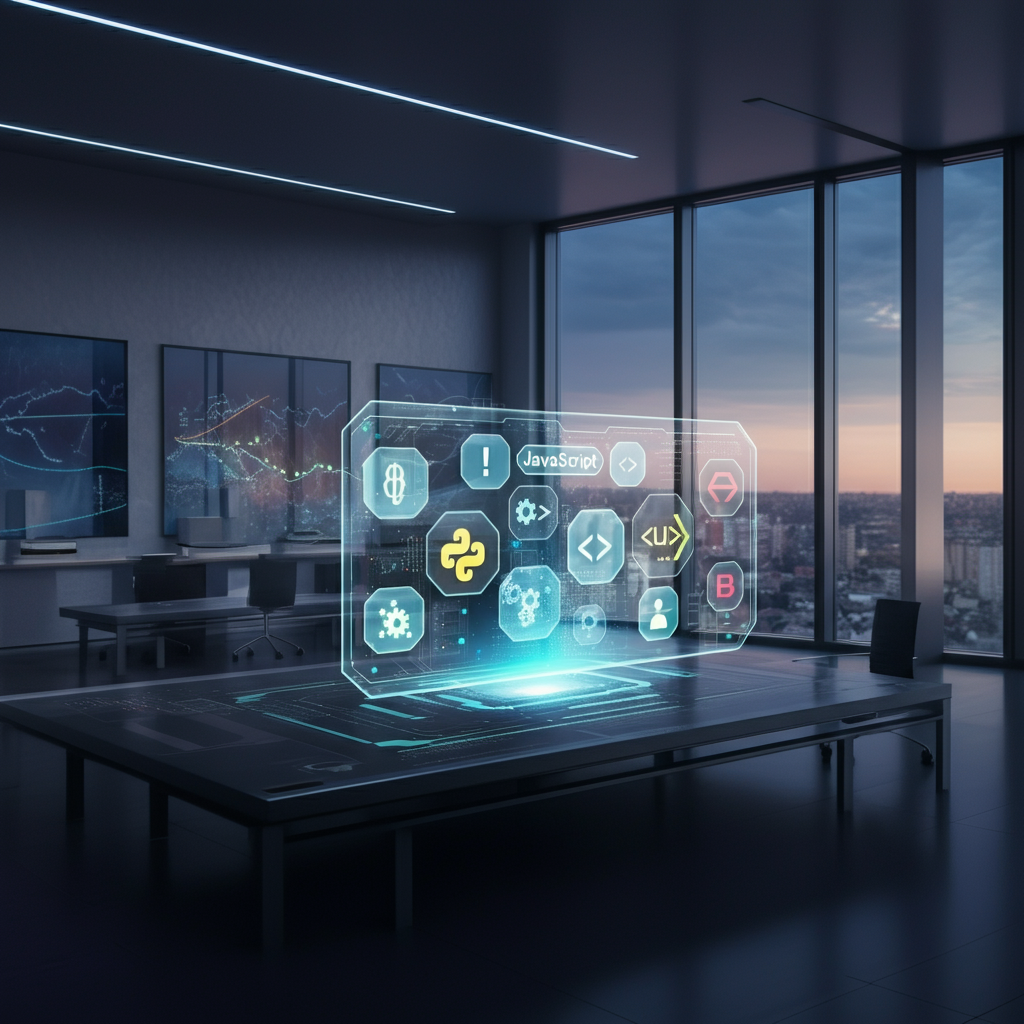
Introduction to Predictive Analytics
Navigating the complexities of today’s business world requires more than intuition and traditional methods. Predictive analytics emerges as a critical tool, enabling companies to forecast future trends by analyzing both current and historical data. This approach utilizes advanced statistical techniques, machine learning algorithms, and data mining processes to uncover patterns that can inform future decisions.
In practice, predictive analytics involves a combination of data collection, analysis, and model building. The process begins with gathering relevant data from various sources, which could include sales records, customer feedback, and market reports. This data is then processed and analyzed to identify patterns and correlations. By leveraging these insights, businesses can make predictions about future events, such as consumer behavior changes, market demands, and potential risks.
One key aspect of predictive analytics is its reliance on sophisticated models that can handle complex datasets. These models, often powered by AI, can manage vast amounts of information more efficiently than traditional methods. For example, machine learning algorithms can adapt to new data inputs, continually refining their predictions as more information becomes available. This adaptability makes predictive analytics a powerful asset for businesses looking to stay ahead in rapidly changing markets.
Another important facet is the role of data visualization in making sense of predictive analytics. Tools that convert raw data into visual formats like charts and graphs enable stakeholders to quickly grasp key insights and trends. This visual representation aids in better understanding and communicating the findings, facilitating data-driven decision-making across all levels of an organization.
By incorporating predictive analytics into their strategies, companies can optimize various aspects of their operations. From improving supply chain efficiency to enhancing customer experience, the applications are vast and varied. The ultimate goal is to transform data into actionable insights, allowing businesses to make informed decisions that drive growth and innovation.
The Role of AI in Gathering Data
AI technologies have significantly changed how businesses collect and manage data. With the ability to process large volumes of information quickly and accurately, AI tools streamline data collection from a variety of sources such as customer interactions, sales records, and social media. This ensures that businesses have access to comprehensive and up-to-date information, providing a strong foundation for predictive analytics.
One of the standout features of AI in data gathering is its ability to filter out irrelevant information, focusing on data that provides real value. Machine learning algorithms are particularly adept at identifying pertinent data points, reducing the time and effort required to sift through massive datasets. These algorithms can identify patterns and anomalies that might be missed by traditional data collection methods, enhancing the overall quality of the data.
Moreover, AI-powered tools facilitate real-time data updates, allowing businesses to keep pace with fast-evolving market conditions. This real-time capability is crucial for making timely decisions, especially in industries where market dynamics can change rapidly. Businesses no longer have to rely on outdated reports or delayed data inputs; instead, they can access current information that reflects the latest market trends and consumer behaviors.
Another critical aspect is the reduction of human errors and biases in data collection. AI systems operate based on programmed algorithms, minimizing the risk of inaccuracies that can arise from manual data entry or subjective interpretations. This leads to a higher level of data integrity, which is essential for making reliable predictions.
AI also enhances data integration, allowing businesses to combine information from various sources into a unified dataset. This holistic view is invaluable for comprehensive analysis, providing deeper insights into market trends and customer preferences. The ability to integrate and analyze diverse data types, such as text, images, and numerical data, further broadens the scope of insights that can be derived.
Overall, AI technologies are revolutionizing the way businesses gather and process data, making the entire process more efficient, accurate, and insightful.
AI Models for Forecasting Trends
Artificial Intelligence has revolutionized the way businesses forecast trends, using sophisticated models that learn from vast datasets. Among the most effective models are neural networks, decision trees, and support vector machines. These models excel at identifying intricate patterns within data, offering insights that are more nuanced and actionable than those derived from traditional methods.
Neural networks, inspired by the human brain’s structure, consist of layers of interconnected nodes that process information. They are particularly adept at handling large volumes of data and can identify complex relationships between variables. This capability makes them ideal for predicting consumer behaviors, market trends, and even financial fluctuations.
Decision trees offer another powerful approach, simplifying decision-making processes by breaking down data into smaller, manageable parts. Each “branch” of the tree represents a decision rule, and each “leaf” signifies an outcome. This structure makes it easier to understand the logic behind predictions, providing clear and actionable insights for business leaders.
Support vector machines, on the other hand, are excellent for classification and regression tasks. They work by finding the optimal boundary that separates different classes in the data. This makes them highly effective for tasks such as fraud detection, risk assessment, and customer segmentation.
One of the standout features of these AI models is their ability to continually improve. Unlike traditional models that rely on static algorithms, AI models dynamically update their parameters as new data becomes available. This ongoing learning process ensures that predictions remain accurate and relevant, even in rapidly changing market conditions.
These advanced models are not only more accurate but also more efficient, capable of processing and analyzing data at unprecedented speeds. This efficiency allows businesses to make timely, data-driven decisions, staying ahead of competitors and adapting swiftly to new trends. The adoption of these AI models marks a significant leap forward in the realm of business forecasting.
Benefits of AI in Business Forecasting
AI integration in business forecasting brings about substantial improvements in accuracy and operational efficiency. In the retail sector, for instance, predictive analytics helps fine-tune inventory management by precisely forecasting product demand. This reduces the costs related to holding surplus stock and prevents situations like stockouts or overstocking, enhancing inventory management and reducing associated costs by enhancing inventory management and reducing associated costs..
AI-driven insights also significantly boost decision-making processes by minimizing guesswork and providing data-driven guidance for strategic planning to improve strategic planning with data-driven insights.. In financial services, AI can predict market trends and economic shifts, enabling more accurate risk assessments and investment strategies. By analyzing historical data and identifying patterns, AI tools provide financial analysts with robust models that improve forecasting accuracy.
In marketing, AI enhances customer segmentation and targeting. By analyzing consumer behavior data, businesses can develop personalized marketing strategies that increase engagement and conversion rates. AI tools can predict which customers are likely to respond to specific campaigns, allowing for more efficient allocation of marketing resources.
Moreover, AI’s ability to process and analyze large volumes of data in real-time allows businesses to stay agile. In manufacturing, for example, AI can predict equipment failures, thereby reducing downtime and maintenance costs. This proactive approach helps maintain production schedules and improves overall operational efficiency.
AI also plays a vital role in enhancing customer service. Predictive models can anticipate customer needs and preferences, allowing businesses to offer more personalized experiences. This not only improves customer satisfaction but also builds brand loyalty and drives long-term growth.
Integrating AI into business forecasting provides a competitive edge, enabling companies to anticipate changes, respond swiftly, and make well-informed decisions that drive success.
Challenges and Solutions
While AI has revolutionized trend forecasting, it does come with its own set of challenges. One significant issue is integrating AI systems into existing business operations. Organizations often encounter obstacles related to data privacy, system compatibility, and the necessity for specialized skills to manage these advanced technologies.
To tackle the data privacy concern, companies must ensure that they comply with regulations like GDPR and CCPA, which mandate stringent data protection measures. This might involve adopting encryption methods, securing data storage, and regularly auditing data usage practices to safeguard sensitive information.
System compatibility can also be a roadblock, as legacy systems may not seamlessly integrate with modern AI platforms. This issue can be addressed by conducting a thorough assessment of current IT infrastructure and identifying the gaps that need to be filled. Utilizing middleware solutions can act as a bridge between old and new systems, ensuring smoother integration.
Another significant challenge is the skills gap within the workforce. AI technologies require a unique blend of skills that traditional business roles might not cover. Investing in employee training programs focused on data science, machine learning, and AI applications is essential. Additionally, hiring experts with specialized knowledge can provide the necessary expertise to manage and optimize AI systems.
Scalability is another concern. As businesses grow, the volume of data and the complexity of AI models also increase. To manage this, cloud-based AI solutions can offer scalable resources that adjust according to the organization’s needs, making it easier to handle large datasets and complex computations.
Addressing these challenges requires a strategic approach that includes investing in technology, training, and infrastructure. By doing so, businesses can unlock the full potential of AI, ensuring that they can efficiently and effectively leverage advanced predictive analytics for trend forecasting.
The Future of AI in Trend Forecasting
Looking ahead, AI will continue to revolutionize trend forecasting with breakthroughs in deep learning, natural language processing, and edge computing. Deep learning algorithms, which excel at analyzing complex, high-dimensional data, will offer even more precise forecasts by understanding intricate patterns and relationships within datasets. Natural language processing will enhance AI’s ability to interpret and analyze text-based data, such as customer reviews, social media posts, and news articles, providing richer insights into consumer sentiment and emerging trends.
Edge computing, which processes data closer to its source, will further boost AI’s predictive capabilities. By reducing latency and enabling real-time analysis, edge computing will allow businesses to respond instantly to market changes and operational shifts. This will be particularly valuable in industries where timely decisions are crucial, such as finance, healthcare, and logistics.
The integration of AI with Internet of Things (IoT) devices will also play a pivotal role in future trend forecasting. IoT devices generate vast amounts of data, offering real-time insights into consumer behavior, supply chain dynamics, and operational performance. Combining this data with AI-driven analytics will provide businesses with a comprehensive view of their environment, enabling more accurate and timely forecasts.
Moreover, advancements in AI explainability will make AI models more transparent and easier to understand. This will help business leaders gain confidence in AI-driven insights, fostering broader adoption and integration into strategic planning. As AI continues to evolve, we can expect it to become an indispensable tool in forecasting business trends, helping companies stay agile and competitive in an ever-changing landscape.
Conclusion
The integration of AI into business forecasting marks a transformative shift in how organizations predict and respond to market dynamics. By utilizing advanced algorithms and machine learning models, companies can derive actionable insights from vast datasets, allowing for more precise and strategic planning. This not only improves operational efficiency but also enhances decision-making processes across various sectors, from retail and finance to marketing and manufacturing.
One of the standout benefits of AI-driven forecasting is its ability to process and analyze data in real-time. This capability ensures that businesses can stay ahead of market trends, making timely adjustments to their strategies. Additionally, AI’s proficiency in reducing human error and biases further elevates the reliability of the forecasts, offering a higher degree of confidence in the decisions made.
Moreover, the scalability of AI solutions means that businesses of all sizes can leverage these technologies to gain a competitive edge. Whether it’s optimizing inventory management, predicting equipment failures, or personalizing customer interactions, the applications are both extensive and impactful.
While the adoption of AI does come with its set of challenges, such as data privacy concerns and the need for specialized skills, the solutions to these issues are well within reach. By investing in proper training, robust infrastructure, and compliance measures, organizations can effectively integrate AI into their forecasting processes.
Looking forward, advancements in AI technologies like deep learning and natural language processing will further enhance predictive capabilities, providing even more nuanced insights. Businesses that embrace these innovations will be better positioned to navigate the complexities of modern markets, ensuring sustained growth and success in an ever-evolving landscape.