Manufacturing efficiency is key for businesses to stay ahead. AI can help a lot in this area. It makes processes smoother, cuts costs, and boosts product quality.
AI changes the game for the manufacturing world. It lets companies quickly meet market and customer needs.
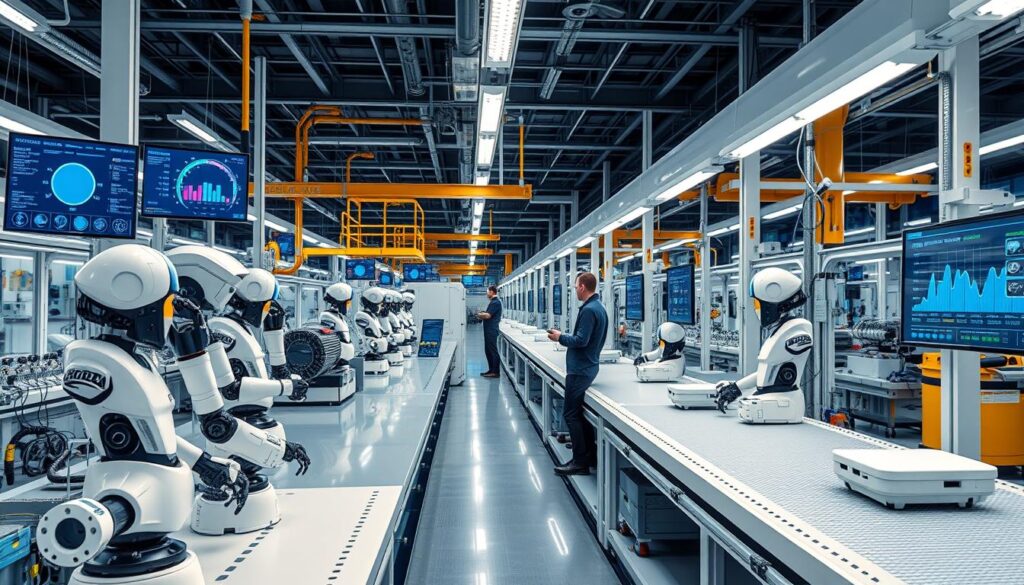
AI optimizes production, predicts when things need fixing, and checks quality. This saves money and boosts work speed. As the industry grows, using AI will be more and more important.
Key Takeaways
- AI can help manufacturers optimize production processes and improve manufacturing efficiency
- AI applications can predict maintenance needs and reduce downtime
- AI can improve quality control and reduce product defects
- The adoption of AI can lead to significant cost savings and increased productivity
- AI will play a crucial role in the future of manufacturing, enabling companies to respond quickly to changing market demands
- Manufacturers can benefit from AI by streamlining their processes and improving product quality
The Evolution of AI in Modern Manufacturing
Manufacturing has changed a lot with the help of artificial intelligence (AI). It moved from old ways to new, AI-based methods. This change is part of industry 4.0, focusing on using automation and data in making things.
Now, more companies want to use AI to make their production better. They see AI as a way to save money and make products of higher quality. The trend shows that AI use in making things will grow a lot in the coming years.
Traditional Manufacturing vs AI-Enhanced Production
Old ways of making things are being replaced by new AI-based methods. These new methods are more efficient and cost less. Here are some main differences:
- Automated production processes
- Predictive maintenance and quality control
- Real-time data analysis and decision-making
Key Drivers of AI Adoption in Manufacturing
Several things push companies to use AI in making things. They want to work better, spend less, and make products that are better. Also, having lots of data and new AI tech helps in this growth.
Current Market Statistics and Trends
There’s a big need for AI solutions in making things. AI use in making things is set to grow a lot in the next few years. It will focus on things like fixing things before they break, checking product quality, and making supply chains better. Here are some important stats and trends:
Year | AI Adoption Rate | Industry 4.0 Investment |
---|---|---|
2022 | 20% | $10 billion |
2023 | 30% | $15 billion |
2024 | 40% | $20 billion |
Understanding the Core Components of Manufacturing AI
Manufacturing AI uses key parts like AI components, machine learning, and data analytics. These work together for smart manufacturing. They help with predictive maintenance, quality control, and better supply chains.
AI and machine learning help analyze data from many sources. They find patterns and guide decisions. Data analytics gives insights into how things are made. It helps make operations better.
Some main uses of manufacturing AI are:
- Predictive maintenance: uses machine learning to forecast when equipment might fail and plan for upkeep.
- Quality control: uses data analytics to watch over production quality and spot any issues.
- Supply chain optimization: uses AI to make supply chain work better and cut costs.
Knowing about manufacturing AI’s core parts helps companies use AI, machine learning, and data analytics. This way, they can improve their work and stay ahead in the market.
AI Applications in Manufacturing: Boosting Efficiency and Reducing Costs
Artificial intelligence is changing the manufacturing world. It makes things more efficient and cheaper. A big area where AI shines is in predictive maintenance. This lets makers know when equipment might break down, so they can fix it before it happens.
This way, they avoid long stops and work better overall.
Predictive Maintenance Systems
Predictive maintenance systems use smart algorithms to look at data from sensors. They guess when equipment might fail. This lets makers plan when to do maintenance, without stopping production too much.
Quality Control and Inspection
AI helps with quality control and checking products. It uses computer vision and learning to spot problems. This makes products better and cuts down on waste.
AI also helps with supply chain optimization. This makes supply chains better and cheaper. And it helps with planning and scheduling production, making things faster and more efficient.
- Predictive maintenance systems reduce downtime and increase productivity
- AI-enhanced quality control and inspection systems improve product quality and reduce waste
- Supply chain optimization reduces costs and improves efficiency
Smart Factory Implementation Strategies
Starting a smart factory needs careful planning and strategy. It involves adding AI and digital technologies to manufacturing. First, you must assess your current setup and set goals for the smart factory.
Adopting industry 4.0 principles is crucial. These include automation, data exchange, and new manufacturing technologies. They help make the factory more efficient and productive.
Implementing a smart factory should be done in phases. This allows for testing and adjustments. It helps avoid disrupting current operations and makes the transition smoother.
Important technologies in smart factories include predictive maintenance systems, quality control and inspection systems, and supply chain optimization tools. These tools help make the factory more efficient and competitive.
Benefits of smart factory strategies include:
- Improved efficiency and productivity
- Enhanced quality control and inspection
- Optimized supply chain management
- Increased competitiveness in the market
As manufacturers adopt industry 4.0 and smart factory technologies, they see big improvements. A well-planned strategy leads to a more efficient and competitive factory. This keeps them ahead in the fast-changing world of industry 4.0.
Machine Learning in Production Optimization
Machine learning is key in making production better by finding and fixing problems fast. It uses algorithms to look at data from sensors and machines. This helps manufacturers make their processes more efficient, cut down on waste, and make products better.
One big plus of machine learning is how it helps prevent defects. It looks at data to spot problems early and tells makers to fix them. This cuts down on waste and makes products better, saving time and money.
Pattern Recognition in Manufacturing Processes
Seeing patterns is a big deal in machine learning for making things better. It looks at data to find patterns and oddities in how things are made. This info helps make production smoother, reduce waste, and make products better.
Real-time Production Adjustments
Being able to change things up fast is another big plus of machine learning. It looks at data to find where things can be better and makes changes right away. This makes products better, cuts down on waste, and makes things run more smoothly.
Defect Prevention Systems
Systems to stop defects are a big part of machine learning in making things better. It looks at data to find problems before they happen and tells makers to fix them. This makes products better, cuts down on waste, and saves time and money.
- Reduced waste and improved product quality
- Improved overall efficiency and reduced need for rework
- Enhanced production optimization and reduced costs
By using machine learning and defect prevention systems, makers can make their production better, cut down on waste, and make products better. This makes things run more smoothly, saves money, and makes production optimization better.
Robotics and Automated Systems Integration
The use of robotics and automated systems is key in today’s manufacturing. It helps companies make their production better and more efficient. By using robotics and automated systems, tasks are made easier, costs go down, and products get better.
Some benefits of integration include:
- Increased productivity
- Improved product quality
- Reduced labor costs
- Enhanced supply chain management
Companies like Siemens and KUKA are using robotics and automated systems to improve their manufacturing. By adopting these technologies, businesses can stay ahead in the market and succeed in the long run.
To make integration work, robotics and automated systems must match the company’s strategy and goals. This way, businesses can fully use these technologies and see big improvements in their manufacturing.
Technology | Benefits |
---|---|
Robotics | Increased productivity, improved product quality |
Automated Systems | Reduced labor costs, enhanced supply chain management |
Data Analytics and Manufacturing Intelligence
Manufacturing intelligence is key in today’s world, and data analytics is essential for it. By using data analytics, manufacturers can understand their production better. This helps them make smart choices and grow their business.
Data analytics shows where improvements are needed and how to make production better. It also helps predict problems before they happen.
Real-time monitoring is a big part of data analytics in manufacturing. It tracks important production metrics like how much is made, quality, and how well equipment works. This lets manufacturers quickly fix problems, cut downtime, and boost efficiency. Predictive analytics is also crucial. It helps forecast future trends and challenges, helping manufacturers stay ahead.
Some key benefits of data analytics and manufacturing intelligence are:
- Improved production efficiency
- Enhanced product quality
- Predictive maintenance and reduced downtime
- Data-driven decision making
By using data analytics and manufacturing intelligence, manufacturers can reach new heights of efficiency and innovation. They can stay competitive in today’s fast world. With predictive analytics and real-time monitoring, they can make smart choices, grow their business, and reach their goals.
Cost Reduction Through AI Implementation
Using AI in manufacturing can cut costs a lot. It helps by making production more efficient and using less energy and labor. AI also improves product quality and boosts efficiency, saving more money.
Manufacturers should think about the return on investment (ROI) when using AI. The upfront costs can be high, but the long-term gains are worth it. Key considerations include the cost of hardware and software, training, and maintenance, and how AI can make things more efficient.
Direct Cost Savings Analysis
- Reduced energy consumption through optimized production processes
- Lower labor costs through automation and streamlined workflows
- Minimized waste and reduced material costs through predictive maintenance and quality control
Indirect Benefits and ROI
AI also brings indirect benefits like better product quality and happier customers. It helps manufacturers make smart decisions based on data. This can lead to big gains in productivity and revenue.
Implementation Cost Considerations
When planning to use AI, manufacturers need to think about the costs. This includes the cost of hardware and software, training, and any disruptions to production. By weighing these costs against the benefits, manufacturers can make smart choices and get a good return on investment.
Cost Category | Description | Estimated Cost |
---|---|---|
Hardware and Software | Cost of AI systems and infrastructure | $100,000 – $500,000 |
Training and Maintenance | Cost of training personnel and maintaining AI systems | $50,000 – $200,000 |
Disruption to Production | Cost of potential disruptions to production during implementation | $20,000 – $100,000 |
Overcoming Implementation Challenges
Using AI in manufacturing is complex, with many implementation challenges to face. One big issue is change management. AI changes how we work, requiring new processes and workflows. Also, workforce development is key to make sure employees can use AI well.
To tackle these hurdles, manufacturers can follow some steps:
- Make a detailed plan for change management to keep things running smoothly
- Offer training and development for employees to boost their skills
- Have a clear way to communicate with everyone involved in the AI rollout
AI in manufacturing needs careful planning and execution. By tackling implementation challenges and focusing on change management and workforce development, manufacturers can smoothly adopt AI. This leads to better efficiency, productivity, and cost savings.
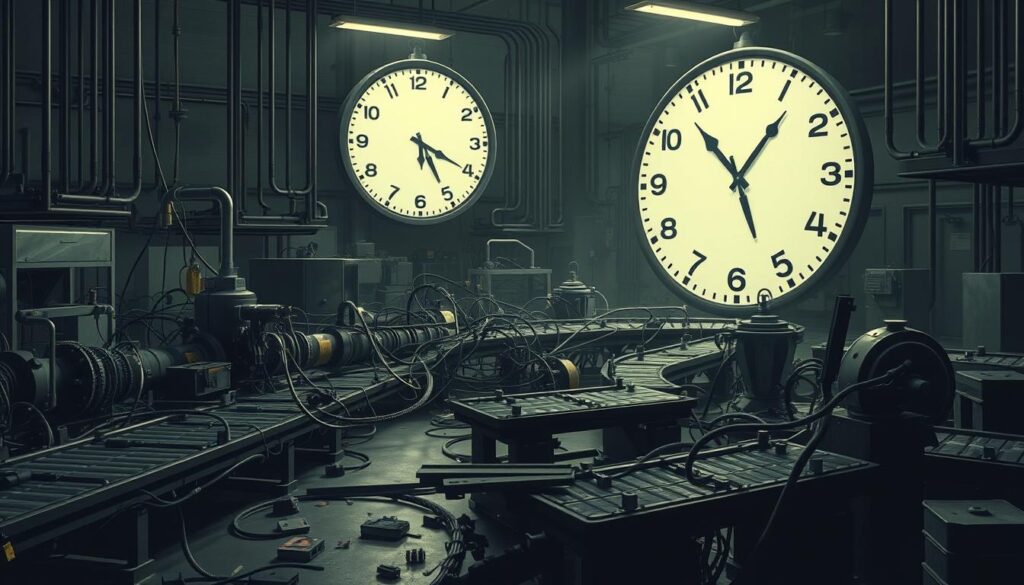
Investing in workforce development and change management helps manufacturers succeed with AI. They can unlock its full potential.
Implementation Challenges | Solutions |
---|---|
Change Management | Develop a comprehensive change management plan |
Workforce Development | Provide training and development programs for employees |
Technological Integration | Establish a clear communication strategy |
Training and Workforce Development
AI is becoming more common in manufacturing, and we need a skilled workforce to work with it. This means focusing on training and developing the skills of current employees. It’s also important to manage changes smoothly for everyone.
To work with AI, employees need skills in data analysis, programming, and thinking critically. Companies can offer training in these areas. This includes learning data analysis, programming languages like Python and R, and improving critical thinking and problem-solving.
Change management is key to helping employees adjust to AI. This includes clear communication, training, and ways to measure success. By investing in training and change management, companies can make sure their employees are ready to use AI and succeed in their jobs.
Some companies have already seen great results from investing in training and change management. For example, a study by the National Institute of Standards and Technology showed that companies that did this saw better productivity and efficiency.
By focusing on training, employee development, and change management, companies can fully use AI in manufacturing. This leads to business success.
Company | Workforce Development Initiative | Results |
---|---|---|
Company A | Employee training program in data analysis | 25% increase in productivity |
Company B | Change management strategy for AI implementation | 30% reduction in errors |
Measuring Success: KPIs and Metrics
To see if AI works well in making things, we need to set up key performance indicators (KPIs) and metrics. These tools help us see if AI is making things better, like making things faster, cheaper, and of higher quality. Success measurement is key to knowing how AI is doing and making smart choices for the future.
Some important KPIs and metrics to look at include:
- Production throughput and efficiency
- Defect rate and quality control
- Supply chain optimization and inventory management
- Cost savings and return on investment (ROI)
By watching these metrics and tweaking the AI, makers can make their production better, cut costs, and make products of better quality.
Checking these KPIs often helps makers fine-tune their AI use. This makes sure it fits with their business aims and goals. This way, they can get the most out of AI and succeed in making things for a long time.
Metric | Description |
---|---|
Production Throughput | Measures the quantity of products manufactured within a given time frame |
Defect Rate | Measures the number of defective products produced |
Cost Savings | Measures the reduction in costs achieved through AI implementation |
Future Trends in Manufacturing AI
The manufacturing world is about to change a lot. This change comes from emerging technologies like artificial intelligence, machine learning, and the Internet of Things (IoT). These technologies will be key in shaping the industry’s future trends.
Some important areas to keep an eye on include:
- Advancements in machine learning and predictive analytics
- Increased adoption of IoT devices and sensors
- Growing focus on sustainable manufacturing practices
As the industry grows, we’ll see big steps forward in emerging technologies. This will lead to better efficiency, more productivity, and less harm to the environment. By adopting these future trends and focusing on sustainable manufacturing, companies can thrive in a fast-changing world.
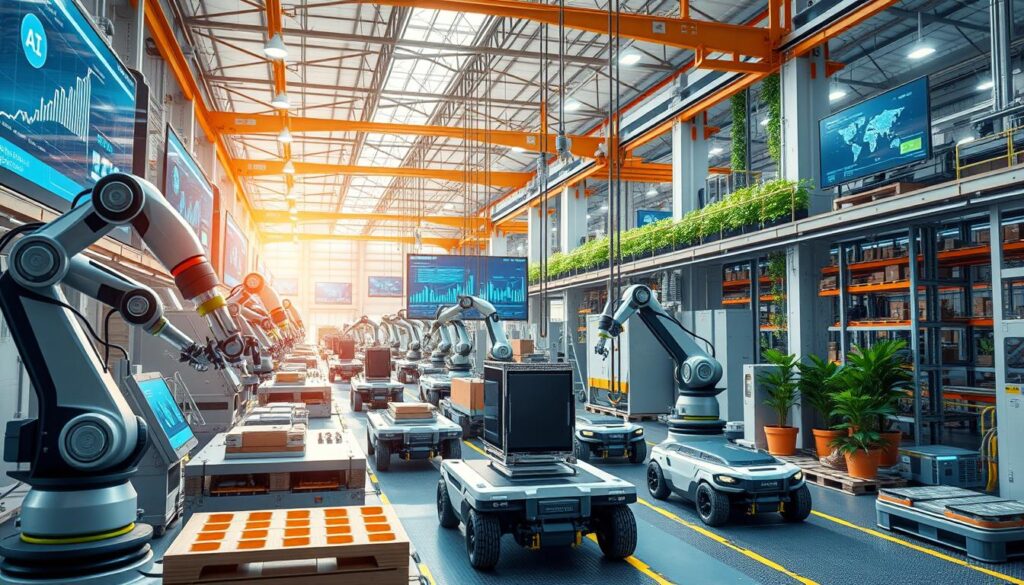
Conclusion
As we wrap up our look at AI’s big impact on manufacturing, it’s clear the future is bright. AI has already made things more efficient, cut costs, and helped make production more sustainable. This shows how powerful AI can be in the industry.
Companies that get AI right are leading the way in Industry 4.0 and more. They’re using smart factories, machine learning, and robots to change the game. These technologies are key to shaping the future of making things.
But, there are still hurdles to overcome, like getting AI to work and training workers. Yet, the benefits of using AI in making things are huge. As we move towards Industry 5.0 and focus more on being green, AI will play a big role. It will help us innovate, make better decisions, and stay ahead of the competition.
The future of making things is all about AI. Companies that invest in these new technologies will be ready for the changing world. They’ll be the leaders in their markets.
FAQ
What are the key components of manufacturing AI?
The main parts of manufacturing AI are machine learning, data analytics, and the Internet of Things (IoT). These technologies help make manufacturing smarter. They enable things like predictive maintenance, quality control, and better supply chain management.
How can AI boost efficiency and reduce costs in manufacturing?
AI makes manufacturing more efficient in several ways. It helps with predictive maintenance to cut downtime. It also improves quality control and optimizes supply chains and production planning. These efforts lead to cost savings and better efficiency.
What are the key strategies for successful smart factory implementation?
To set up a smart factory, you need a solid plan. Start by assessing your current setup and setting clear goals. Then, roll out new technologies in phases. It’s also important to manage change and train your workforce for a smooth transition.
How can machine learning optimize production processes?
Machine learning is key in making production better. It recognizes patterns, makes adjustments in real-time, and prevents defects. These actions boost efficiency, cut waste, and improve product quality.
What are the key challenges in overcoming AI implementation in manufacturing?
Implementing AI in manufacturing comes with challenges. These include managing change, training workers, and integrating technology. To overcome these, focus on clear communication, employee training, and a step-by-step approach to AI adoption.
How can manufacturers measure the success of their AI implementation?
To gauge AI success, use key performance indicators (KPIs) like efficiency gains, cost savings, and quality improvements. Set these metrics early and track them regularly. This helps understand AI’s impact and guides future development.
What are the future trends in manufacturing AI?
The future of manufacturing AI looks exciting. New technologies like advanced machine learning and robotics will emerge. There will also be a shift towards Industry 5.0 and sustainable practices. Staying updated on these trends is crucial for manufacturers to stay ahead.