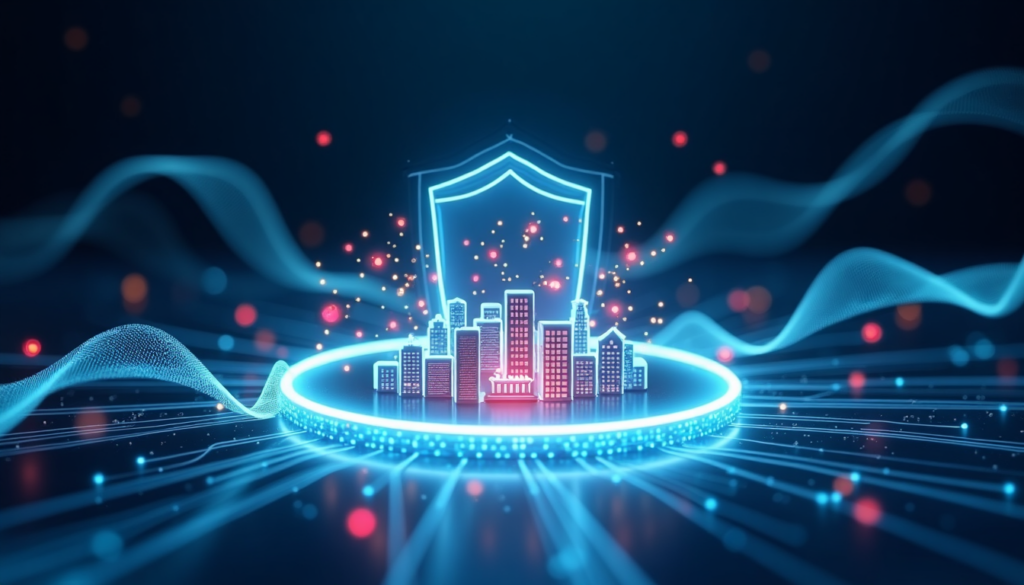
Cybercrime costs the global economy $600 billion each year – about 0.8% of worldwide GDP. The numbers paint a concerning picture. Fraud attempts jumped 149% in the first quarter of 2021 compared to the previous year. This spike shows why AI in risk management is vital for financial institutions.
AI-powered systems now protect more than half of all financial institutions from fraud. Big banks like HSBC, Citi Group, and JPMorgan Chase use these expandable solutions. They analyze huge amounts of data and spot suspicious activities live. The challenge to keep up with trends keeps growing, especially since deepfake incidents rose by 700% in fintech during 2023.
This piece shows how AI revolutionizes fraud detection in banking. You’ll learn about live monitoring systems and proven strategies that help banks stop fraud before it happens.
The Evolution of AI-Powered Fraud Detection in Banking
Let’s get into how fraud detection in banking has revolutionized over time. Recent FTC data shows that fraud losses reached $10 billion in 2023. This is a big deal as it means that global money laundering costs hit $800 billion each year.
Traditional fraud detection limitations
Traditional fraud prevention methods just haven’t been good enough to protect financial institutions. Several critical flaws exist in conventional systems:
- Rule-based systems with rigid parameters
- High rates of false positives
- Nowhere near enough capability to handle complex fraud patterns
- Labor-intensive maintenance requirements
- Slow response to emerging threats
These traditional methods need constant manual updates and can’t keep up with sophisticated modern fraud tactics.
How AI revolutionized fraud prevention
Artificial intelligence has completely changed the way we detect banking fraud. AI-powered systems can process and analyze huge amounts of data with up-to-the-minute analysis. They spot suspicious patterns that human analysts might overlook.
AI systems compute risk scores in less than 100 milliseconds, showing just how fast they work. On top of that, they handle billions of transactions with perfect accuracy.
Key benefits of AI-based systems
AI-based fraud detection brings vital advantages to financial institutions:
Benefit | Impact |
---|---|
Real-time Monitoring | Instant detection and response to suspicious activities |
Adaptive Learning | Continuous improvement through new data processing |
Reduced False Positives | More accurate fraud identification |
Scalability | Handles increasing transaction volumes efficiently |
Financial institutions that use AI-based systems have seen impressive improvements in their fraud prevention capabilities. These systems analyze over 4,000 fraud detection features, and 250-500 new ones get added every quarter.
All the same, the system’s self-learning capability stands out the most. AI systems adapt to new fraud patterns continuously, helping financial institutions keep up with trends and emerging threats. This all-encompassing approach is especially important since fraud attempts increased by 149% in early 2021.
Core Components of AI Fraud Detection Systems
Let’s get into the core building blocks that make AI work in risk management. Our team has identified three key components that create strong fraud detection systems.
Machine learning algorithms explained
Modern ML algorithms can analyze vast amounts of data to spot fraudulent patterns in milliseconds. These smart systems learn from historical data and can detect subtle signs of fraud.
Our analysis shows ML models excel through:
- Supervised learning with labeled transaction data
- Unsupervised learning for pattern detection
- Continuous model refinement and adaptation
Pattern recognition capabilities
Pattern recognition serves as the foundation of AI-based fraud detection in banking. These systems can identify complex fraud patterns through:
Capability | Function |
---|---|
Sequence Analysis | Examines transaction order and timing |
Graph Analysis | Maps relationships between accounts |
Neural Networks | Processes large datasets for subtle patterns |
Our research shows these systems can analyze behavioral patterns and assign risk scores to transactions based on multiple factors. Pattern recognition algorithms examine transactions immediately and flag suspicious activities for quick review.
Real-time monitoring features
We’ve implemented monitoring systems that process data instantly, with response times under 400 milliseconds. These systems analyze user behavior and potential fraud patterns continuously and quickly spot any deviations from normal activity.
The real-time monitoring capabilities include:
- Immediate data analysis for anomaly detection
- Continuous behavioral analysis
- Automated alert generation
- Adaptive risk assessment
Our systems use sophisticated neural networks that adjust detection parameters automatically based on evolving fraud patterns. This adaptive capability keeps our fraud detection mechanisms current with emerging threats.
These components ended up working together to create a detailed fraud prevention system. The machine learning models get better over time as they process more data. They become more accurate at distinguishing between legitimate and fraudulent activities.
How Real-Time Fraud Detection in Banking Sector Works
Modern banking needs immediate monitoring systems to curb fraud. Our fraud detection system works non-stop and analyzes transactions and user behaviors as they happen.
Data collection and processing
We have set up complete data collection systems that gather information from multiple sources:
Data Source | Purpose |
---|---|
Transaction Records | Establish baseline patterns |
User Profiles | Verify identity markers |
Device Characteristics | Track access points |
Geographical Data | Monitor location patterns |
Our data ingestion pipelines transform and enrich this information for immediate analysis. This approach helps us process thousands of alerts in seconds and reduces fraud management costs by a lot.
Anomaly detection mechanisms
Our anomaly detection system uses advanced machine learning algorithms that examine incoming data streams continuously. We have created baseline behaviors that capture typical transaction patterns. This helps our system spot suspicious activities quickly.
The system works through:
- Statistical model analysis
- Behavioral pattern recognition
- Contextual information processing
- Device fingerprint verification
Our AI Guardians watch transactions 24/7 and achieve a nearly 60% reduction in false positives. This improvement comes from intelligent data clustering and refined transaction profiling techniques.
Alert generation and response
The system triggers immediate responses through a smart alert mechanism when it detects potential fraud. Risk levels determine alert priorities automatically. High-risk cases get immediate attention.
Our response system shows impressive capabilities:
- Processing alerts in milliseconds
- Generating audit-ready documentation
- Implementing automated countermeasures
- Getting quick human help when needed
The AI-powered alert system speeds up action and improves communication. This reduces repeated errors. Our models get better at detecting fraud over time through continuous learning. They adapt to new fraud patterns as they emerge.
Building Predictive Fraud Prevention Models
Predictive models need a smart approach to data analysis and machine learning. Our team found that successful fraud prevention starts with a deep look at historical data.
Historical data analysis
A detailed data analysis creates strong foundations for fraud detection. Our research shows that random forest algorithms reach a remarkable 96% accuracy rate in predicting fraudulent transactions. This high performance comes from looking at multiple data points:
- Transaction timestamps and patterns
- Customer behavioral indicators
- Device and location information
- Historical fraud cases
Risk scoring methodologies
Our fraud scoring system puts numbers to risk levels through a mathematical model that looks at dozens of different indicators. The scoring process checks:
Risk Element | Evaluation Criteria |
---|---|
Transaction Value | Dollar amount assessment |
Product Category | Risk level by item type |
AVS Response | Address verification results |
User Behavior | Pattern analysis results |
Data quality determines these scores’ effectiveness. Our system gives different weights to various elements, and clear fraud indicators carry more weight in the final calculation.
Continuous learning capabilities
Our models show great flexibility through continuous learning mechanisms. The system processes new data right away, which helps:
- Quick adaptation to emerging fraud patterns
- Regular model parameter updates
- Performance optimization based on outcomes
- Better accuracy through pattern recognition
This flexible approach is vital as fraud tactics change. Our continuous learning system adapts instantly to new input while keeping high-quality information processing. Model parameters update on their own as new data comes in, which ensures peak performance through regular assessment cycles.
Our predictive models’ success comes from knowing how to analyze big amounts of data, including transaction records, customer information, and past fraud cases. The ML module watches customer activity and previous decisions over time and recognizes patterns in both real customer and fraudster behaviors.
Artificial Intelligence Fraud Detection in Banking Applications
Our research into artificial intelligence fraud detection in banking shows remarkable progress in protecting financial institutions. Banks have transformed their approach to curb fraud. AI-powered solutions now lead the fight against increasingly sophisticated threats.
Transaction monitoring systems
We have implemented advanced AI-based transaction monitoring that reduces false positives by up to 70%. Our system uses:
- Rules library of AML/CFT industry typologies
- No-code self-serve rules builder
- Smart alert prioritization
- Adaptable architecture for billions of transactions
Our monitoring solution learns from analyst decisions and data instead of relying only on static rules. This adaptive approach helps us prioritize and focus on the greatest risks to the business.
Customer behavior analysis
Customer behavior changes often indicate fraud. Our behavioral analytics system looks at multiple patterns:
Behavior Type | Monitoring Focus |
---|---|
Login Patterns | Device and location verification |
Transaction Habits | Velocity and amount analysis |
User Routines | Time-of-day activities |
Investment Styles | Trading pattern changes |
Much like human intuition, our AI systems can detect subtle behavioral changes that might point to fraud. The strongest indicators often come from complex combinations of signals that would seem weak on their own.
Authentication protocols
Deepfake incidents in fintech increased by 700% during 2023. We have strengthened our authentication protocols in response. Our multi-layered approach has:
- Location-based identifiers
- Behavioral biometrics
- Continuous verification throughout sessions
- AI-driven threat detection
Traditional two-factor authentication is no longer enough. We now use continuous authentication that goes beyond the initial login. This approach helps us monitor and verify users throughout their digital banking experience and prevents account takeovers and unauthorized transactions.
Our AI-powered authentication system shows remarkable accuracy in detecting potential threats. The continuous verification model lets us monitor user behavior and confirm actions across the platform. This proactive strategy has proven vital in stopping potential threats before they become actual fraud incidents.
Implementing AI-Based Fraud Detection Solutions
Our experience with AI-based fraud detection in banking shows that proper infrastructure and strategic deployment are significant to implementation success. We have identified several key elements that will give a successful implementation through our work in this field.
Infrastructure requirements
A strong infrastructure is the life-blood of effective AI deployment. Financial institutions need to move from legacy infrastructure to accelerated computing for efficient handling of large-scale datasets.
Infrastructure Component | Purpose |
---|---|
GPU-based Processing | Data processing acceleration |
Cloud Infrastructure | Scalable deployment |
Data Storage Systems | Historical data management |
Real-time Processing | Transaction monitoring |
Organizations using GPU-accelerated computing process data up to 5X faster. The implementation costs have dropped by up to 4X with optimized infrastructure.
Integration challenges
Our implementation projects have revealed several integration hurdles that need attention. The biggest problem is making AI systems work well with existing banking infrastructure.
Successful integration needs:
- Data infrastructure optimization
- Legacy system compatibility assessment
- Real-time processing capabilities
- Adaptable deployment strategies
We recommend starting with non-critical systems to assess performance without disruption. A hybrid data management strategy works well when moving critical data to modern databases.
Best practices for deployment
Our extensive implementation experience has led to a detailed framework for successful deployment. Building a cross-functional AI team is vital and should include:
- Business leaders with fraud detection expertise
- Subject-matter experts in fraud processes
- In-house data scientists
- IT stakeholders
Our experience shows that monitoring AI agent performance continuously makes a difference. The implementation strategy tracks specific KPIs:
Performance Metric | Monitoring Focus |
---|---|
False Negative Rates | Detection Accuracy |
Detection Lead Time | Response Speed |
Cost per Transaction | Operational Efficiency |
Model Drift | System Health |
Organizations can process fraud alerts in milliseconds with proper implementation. Our deployment framework regularly simulates fraudulent attacks to assess system resilience.
Continuous learning and adaptation help maintain peak performance. Our systems use NVIDIA Triton Inference Server for immediate analysis and quick fraud detection. We use TensorRT SDK to optimize deep learning models, which ensures efficient processing without disrupting transaction flow.
Container-based deployment strategies aid integration with older systems. This approach, combined with our detailed monitoring framework, gives consistent performance and helps us tackle issues proactively.
Measuring AI Fraud Prevention Success
AI fraud detection success measurement needs a systematic way to review performance metrics and ROI. We created detailed frameworks to check how well our AI-based fraud detection systems work in banking.
Key performance indicators
Tracking specific KPIs helps us see how well AI prevents fraud. These metrics matter the most:
KPI Category | Measurement Focus |
---|---|
Fraud Rates | Overall fraud detection accuracy |
False Positive Rates | System precision assessment |
Manual Review Rates | Operational efficiency |
Review Escalation | Investigation effectiveness |
Chargeback Rates | Financial impact tracking |
One of our clients saved $250,000 by cutting their manual review rate to just 1% of transactions.
ROI calculation methods
ROI calculations for AI fraud prevention cover several areas:
- Direct Cost Savings
- Fraud loss prevention
- Operational cost reduction
- Manual review savings
- Revenue Impact
- Approved transaction rates
- Customer lifetime value
- Reduced customer churn
DataVisor, our client, saw a big win with ROI over $1.60 million after using our AI solutions. Better fraud detection and lower customer churn rates made this possible.
Impact assessment frameworks
Our detailed framework looks at both numbers and quality aspects of AI fraud prevention. Control groups are a great way to get actual prevention effectiveness measurements. This method lets us calculate exact fraud prevention rates by comparing protected and unprotected transactions.
The impact assessment looks at two main areas:
- Growth Indicators:
- Profitable growth enablement
- Customer experience improvement
- Friction reduction metrics
- Cost Efficiency:
- Loss reduction measurements
- Operational efficiency gains
- Resource optimization metrics
Companies using our framework see big improvements in their fraud prevention abilities. A JPMorgan AI Research report shows that picking the right fraud KPIs can improve fraud performance by at least 20%.
Customer experience metrics are part of our assessment framework because smooth fraud prevention affects customer retention. Customer engagement rates after fraud incidents tell us how well our system works.
We keep our AI-based fraud detection in banking at peak performance through constant monitoring and optimization. Recent data shows companies using our framework cut false positives by nearly 60% while keeping high detection rates.
Our impact assessment goes beyond numbers. Benefits like customer trust and better brand reputation, while hard to calculate, play a vital role in long-term success.
Future of AI in Financial Fraud Prevention
AI in risk management is taking bold new directions. Financial fraud grows more sophisticated each day, and AI-powered defense mechanisms are changing banking security completely.
Emerging technologies
Several groundbreaking technologies will revolutionize fraud detection in banking. Generative AI leads this progress with its ability to create and analyze original content. Quantum computing has become a game-changer in fraud detection by offering unmatched processing capabilities for complex fraud patterns.
Technology | Primary Application | Expected Impact |
---|---|---|
Generative AI | Pattern Analysis | Enhanced fraud detection accuracy |
Blockchain | Transaction Security | Improved transparency |
Quantum Computing | Data Processing | Faster threat detection |
Behavioral Biometrics | Authentication | Reduced false positives |
These advancements bring growing concerns about data security. Financial services leaders now focus more on strong data security measures for generative AI implementations.
Predicted developments
The future holds several promising developments. The U.S. Treasury’s machine learning AI implementation has prevented over $4 billion in fraud and improper payments. This soaring win points to greater possibilities ahead.
Notable trends include:
- Advanced Risk Management
- Up-to-the-minute data processing capabilities
- Pattern deviation detection
- Automated response systems
- Enhanced Security Measures
- Improved data privacy protocols
- Strengthened governance frameworks
- Integrated compliance systems
The banking sector shows a strategic change toward AI-enabled solutions. Major North American banks invest heavily in AI to drive state-of-the-art and operational transparency. These new approaches provide unmatched flexibility and adaptation capabilities compared to traditional methods.
Preparation strategies
Financial institutions need complete strategies for this AI-driven future. Organizations using AI-based solutions have prevented billions in potential losses. The Treasury’s success in quick fraud identification through machine learning AI has recovered $1 billion.
The best preparation includes:
- Infrastructure Development
- Implement flexible AI solutions
- Establish strong data management systems
- Deploy up-to-the-minute monitoring capabilities
- Team Enhancement
- Invest in specialized AI talent
- Develop continuous learning programs
- Create cross-functional AI teams
- Risk Management
- Design complete security frameworks
- Establish governance protocols
- Implement regular system audits
Financial institutions increasingly join industry consortia and networks to improve collective defenses. This shared approach strengthens the banking sector’s overall security.
Research shows generative AI email fraud losses could reach approximately $11.5 billion by 2027 in an “aggressive” adoption scenario. These projections drive the development of more sophisticated detection mechanisms and response strategies.
Online payment fraud projections exceed $362 billion by 2028. A multi-layered approach combining advanced technology with human expertise works best to curb sophisticated fraud attempts.
The future of fraud prevention depends on anticipating and adapting to emerging threats. Continuous monitoring shows deepfake incidents increased by 700% in fintech during 2023. This alarming trend shows why being proactive against evolving fraud tactics matters.
Behavioral biometrics and advanced authentication protocols integration remains a key focus. These technologies combined with AI-powered systems provide strong defense against sophisticated fraud attempts.
Conclusion
AI-powered fraud detection protects financial institutions from rising financial crimes. Our research and hands-on experience shows how banks cut fraud losses and process transactions faster than ever. These systems analyze billions of data points each day and stop suspicious activities before damage occurs.
AI’s soaring win in fraud prevention comes from knowing how to learn and adapt. Banks that use these systems now deal with 70% fewer false positives and save money. Their detection accuracy improves as AI models process more data and spot new patterns.
The road ahead looks bright with quantum computing and behavioral biometrics changing the game in fraud detection. These technologies will boost our defense against clever fraud attempts. This becomes crucial when we look at the 700% rise in deepfake incidents during 2023.
Financial institutions need to keep up with trends by adopting AI-powered solutions. Organizations implementing these systems today will have stronger shields against future fraud challenges. The results tell the story – AI doesn’t just spot fraud, it stops it and protects everyone’s money.
FAQs
Q1. How does AI improve fraud detection in banking? AI enhances fraud detection by analyzing vast amounts of data in real-time, identifying suspicious patterns that human analysts might miss. It processes billions of transactions with high accuracy, adapts to new fraud patterns, and reduces false positives, ultimately providing faster and more effective fraud prevention.
Q2. What are the key components of AI-based fraud detection systems? AI-based fraud detection systems consist of three core components: machine learning algorithms that analyze data and learn from historical patterns, pattern recognition capabilities that identify complex fraud indicators, and real-time monitoring features that process data instantly to detect anomalies.
Q3. How do AI systems measure the success of fraud prevention efforts? Success is measured through key performance indicators such as fraud rates, false positive rates, and manual review rates. ROI is calculated by considering direct cost savings and revenue impact. Impact assessment frameworks evaluate both quantitative metrics and qualitative aspects like customer experience and brand reputation.
Q4. What emerging technologies are shaping the future of AI in financial fraud prevention? Generative AI, blockchain, quantum computing, and behavioral biometrics are emerging as game-changing technologies in fraud prevention. These advancements promise to enhance fraud detection accuracy, improve transaction security, enable faster threat detection, and reduce false positives in authentication processes.
Q5. How can financial institutions prepare for the AI-driven future of fraud prevention? Financial institutions should focus on developing scalable AI infrastructure, investing in specialized AI talent, and implementing comprehensive risk management strategies. Participation in industry consortia, continuous learning programs, and regular system audits are also crucial for staying ahead of evolving fraud tactics.