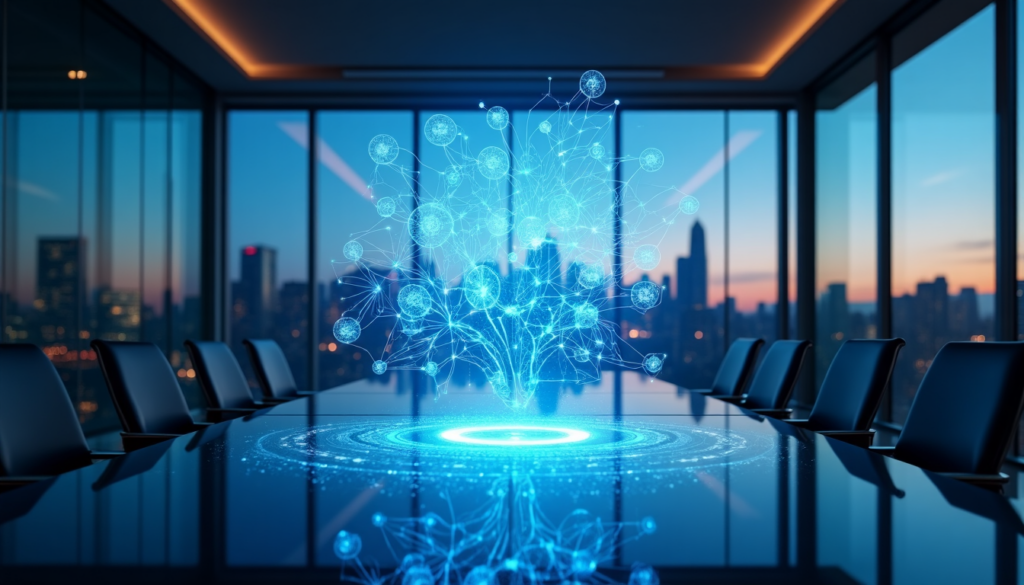
Most of us know that moment – we’re sitting in a meeting while AI terms bounce around the room, leaving us wondering what they actually mean. Machine learning, neural networks – the AI vocabulary can sound like a different language to many business leaders.
AI keeps altering the map of modern business terminology. A solid grasp of these AI terms is a vital part of effective leadership. This detailed guide will help you direct your way through AI jargon and become skilled at using AI concepts in business settings.
This piece breaks down key AI terms by business function. You’ll find clear, practical explanations that boost your confidence when talking about AI technologies – whether you lead strategic decisions, manage operations, or oversee digital transformation.
Foundational AI Concepts for Business Leaders
AI concepts in business don’t have to be complicated. You don’t need a technical background to understand AI terminology – it’s about learning how these tools can improve our business decisions.
Core AI terminology simplified
AI systems can perform tasks that typically need human intelligence. Think of AI as an executive assistant who excels at processing data but still needs your guidance for important decisions.
Here’s a breakdown of the main AI types:
- Narrow AI (Task-Specific): The type we currently use in business – designed for specific tasks like virtual assistants and recommendation systems
- General AI: A theoretical future capability where machines could perform any intellectual task that humans can do
- Machine Learning: A subset of AI that learns from data and improves over time
- Deep Learning: Advanced pattern recognition using neural networks
Business impact of AI technologies
AI adoption has reached unprecedented levels across industries. Recent data shows that 81% of business leaders agree that AI is vital to stay competitive. The global AI market reached USD 62.00 billion in 2020 and will grow at an annual rate of 40.2% through 2028.
The numbers tell an interesting story – 84% of C-suite executives believe AI will help them achieve their growth objectives. This belief has merit, as 92% of large companies report returns on their AI investments.
Key AI capabilities and limitations
Our work with business leaders shows that AI excels in several areas:
- Data Analysis: AI processes vast amounts of information quickly and turns data into applicable information
- Process Automation: Streamlines routine tasks and improves operational efficiency
- Predictive Analytics: Forecasts trends and helps with strategic planning
AI has its limitations. Human oversight remains vital to prevent biases and ensure ethical use. AI can bridge certain skill gaps by providing access to capabilities like coding and translation. Yet it creates new skill requirements that businesses don’t deal very well with.
AI works best as a tool to increase human capabilities rather than replace them. The most successful implementations start with small steps and focus on specific business needs instead of complete transformations.
AI Terms for Strategic Decision Making
The business world driven by data shows how strategic decision-making evolves through AI. Leaders make better informed choices about technology investments and strategic direction by understanding specialized AI terms.
Predictive analytics and business intelligence
Predictive analytics and business intelligence (BI) are the life-blood of modern strategic planning. Predictive analytics uses historical data combined with statistical modeling and data mining to make predictions about future outcomes. The business effects are clear:
- Improved Decision Quality: Nearly three-quarters of organizations report their functional KPIs would improve with greater investment in automation and machine learning technologies
- Operational Efficiency: Workflows powered by predictive analytics directly lead to better profit margins
- Risk Management: Companies use these tools to identify both risks and opportunities in their data patterns
Machine learning for strategic planning
Businesses now approach strategic planning differently through machine learning. ML redefines how we create and measure value in business. Companies that use ML for strategic planning see these benefits:
Forward-Looking KPIs: Traditional KPIs often had a retrospective bias, but machine learning makes them more predictive and prescriptive. Strategic measures now serve as data fuel for training algorithms to optimize business processes.
Informed Insights: ML can process big amounts of data at unprecedented speeds to uncover patterns and insights. This capability shines in areas like demand forecasting, where AI analyzes thousands of seemingly unrelated metrics to make accurate predictions.
Risk assessment terminology
AI’s evolution has brought sophisticated vocabulary to risk assessment. Business intelligence supports many functions in an organization, from recruitment and hiring to training and compliance. These key terms describe AI-driven risk assessment:
- Value Learning: The process where AI learns to pursue specific business values and objectives
- Risk Monitoring: Continuous assessment and tracking of particular risks throughout development and deployment
- Impact Assessment: Evaluation of potential positive or negative outcomes of an AI system
AI risk management needs an ecosystem of assessment, assurance, and audit tools. Independent auditing, oversight bodies, ethics review committees, and transparency registers work together to enable monitoring and mitigation of AI-related risks.
Financial Leadership and AI Technology
Financial leaders today see AI transforming our operations and decision-making processes. Data processing already plays a central role in most financial activities, making the financial sector ready to adopt these advances.
AI terms in financial operations
AI in finance combines advanced algorithms with machine learning to reshape data analysis and decision-making. Our financial institutions see better productivity, lower costs, and improved regulatory compliance. AI proves excellent at processing unstructured data and analyzes complex documents like bond indentures and corporate earnings releases effectively.
Automation and efficiency metrics
Several key metrics help us measure how AI affects financial operations:
- Process Efficiency: AI automates repetitive tasks to cut manual labor costs and optimize workflows
- Error Reduction: Automation leads to fewer errors in financial processes
- Time-to-Complete: Speed comparison between automated and manual processes
- Cost Savings: Tracking of reduced operational expenses through automation
Research shows 98% of institutions believe AI and machine learning can boost their business operations. Yet, 80% to 85% of machine learning projects fail to launch due to logistical and management problems.
Cost-benefit analysis terminology
Both quantifiable and non-quantifiable benefits matter when reviewing AI investments. These principal drivers guide our decisions:
Quantifiable Benefits: AI delivers clear efficiency gains through optimized operations and cost savings. Traditional ROI calculations and payback periods help us measure these benefits.
Strategic Value: Three critical areas deserve our attention:
- Agility: Knowing how to adapt quickly to market changes
- Competitiveness: Long-term advantages over competitors
- Effectiveness: Better business outcomes that prove harder to calculate
Experience teaches us that many AI tools might not show convincing ROI based on efficiency gains alone. Their strategic importance often justifies investment through dedicated “strategic investment” budgets. This helps us balance immediate returns with long-term value creation.
AI affects our financial operations of all sizes, from back-office tasks to customer-facing interfaces. The technology excels at detecting fraud and improves price discovery across asset classes.
Marketing and Customer Experience AI Terms
AI continues to reshape our understanding and connection with customers in marketing departments. Marketing teams now have powerful new ways to analyze, predict, and personalize customer interactions thanks to the rise of AI terminology.
Customer analytics terminology
Modern customer analytics depends on AI-powered segmentation and analysis. Machine learning now identifies patterns in customer data, campaign results, and transaction information beyond simple demographics. Our teams achieve more precise targeting and better business outcomes with these approaches.
Key analytics concepts we use include:
- Lookalike modeling: Using ML to match existing customers with potential new ones
- Customer sentiment analysis: Understanding emotions and opinions in customer interactions
- Business experimentation: Testing ideas through A/B testing and multivariate approaches
AI-powered marketing concepts
AI continues to boost marketing capabilities in remarkable ways. Recent data reveals that 81% of respondents prefer personalized marketing experiences. Our marketing operations rely on several AI-powered approaches:
Data-Driven Decision Making
- Live insights for campaign optimization
- Predictive analytics for customer behavior
- Automated content optimization
Companies that utilize AI in marketing achieve faster revenue growth than their competitors by building closer consumer relationships. This matches our discovery that 71% of consumers now expect personalized interactions.
Personalization technology terms
Personalization stands at the heart of modern marketing strategy. The technology continues to advance across several dimensions:
Core Capabilities:
- Dynamic Content: Live content adaptation based on user behavior
- Predictive Personalization: Using AI to anticipate customer needs
- Cross-channel Optimization: Consistent personalization across all touchpoints
The numbers tell the story – 89% of businesses now invest in personalization as a critical strategy. Our teams deliver more relevant experiences by analyzing past interactions, purchase history, and social media activity.
Our AI-powered personalization creates “intelligent experiences.” These experiences use live data analysis to provide hyper-personalized interactions that substantially increase conversion rates.
Operations and Process Automation Vocabulary
Our teams are adopting AI terminology faster as automation and process optimization become vital to business success. AI-powered solutions have changed the way we manage workflows and quality control.
Workflow automation terms
Workflow automation has grown beyond simple task management. Smart automation systems now work with human input. These systems handle administrative work and flag situations that need human expertise. Modern workflow automation covers:
- Business Process Automation (BPA): Focuses on multi-step processes requiring human involvement
- Digital Process Automation (DPA): Specializes in system-to-system interactions
- Hyperautomation: Goes beyond simple task automation to enable complex, end-to-end process automation
Process optimization concepts
Process mining helps us improve our operations. This technology looks at event logs from enterprise systems and creates virtual views of business processes. Our experience shows that systematic improvements lead to better efficiency.
The best results come from well-arranged and optimized workflows. Successful process optimization needs these key steps:
- Process Mining: Analyzing system data to understand current workflows
- Workflow Management: Creating better employee experiences and improving efficiency
- Continuous Monitoring: Using KPIs to track and improve performance
Intelligent automation combines RPA, AI, and machine learning to create customized process designs that work in organizations of all sizes. This method helps us achieve “process excellence” – where we measure and improve processes continuously.
Quality control terminology
AI-powered systems have changed quality control by detecting and preventing problems before they affect operations. We use advanced technologies that blend machine learning with traditional quality control methods. Our experience proves that AI can analyze sensor data and maintenance records to predict equipment failures.
AI-powered quality control systems are great at spotting patterns and unusual events. These systems process big amounts of data instantly, which helps maintain product quality and reduces manual inspection needs. Our systems find unusual patterns and problems faster than traditional methods.
AI-powered visualizations and algorithms detect product defects better than human inspectors. They often find why problems happen when they might go unnoticed otherwise. This has substantially improved our ability to keep consistent quality standards in our operations.
HR and Talent Management AI Terms
AI terminology and tools are changing how we handle the human side of business. Artificial intelligence helps us manage talent better with evidence-based decisions while keeping the essential human element that HR needs.
Recruitment automation terminology
AI-powered recruitment tools have changed our hiring processes completely. Our experience shows AI helps us fill positions fast, even temporary roles. The recruitment space now has several important technologies:
- AI-Driven Screening: Automated resume prescreening and algorithmic candidate matching
- Interview Intelligence: Tools that analyze and document interview feedback
- Candidate Matching: Systems that analyze qualifications and skills to match with job openings
- Recruitment Chatbots: AI assistants that gather candidate information and conduct preliminary screenings
These tools work best when you have time-saving needs. AI chatbots guide new employees through onboarding, answer questions, and manage document reminders.
Employee analytics concepts
Our HR team uses advanced analytics to understand and improve employee experiences. Recent data shows 76% of HR leaders believe companies must use AI solutions in the next 12-24 months to stay competitive.
Our employee analytics focus on these key metrics:
Analytics Type | Purpose | Impact |
---|---|---|
Engagement Analysis | Monitor employee satisfaction | Makes proactive interventions possible |
Retention Prediction | Identify flight risks | Allows early retention strategies |
Performance Tracking | Measure productivity | Supports evidence-based decisions |
AI helps our teams spot trends in employee behavior and predict which employees might leave. This lets us put targeted retention strategies in place before problems become systemic.
Skills assessment terms
AI-powered skills assessment has changed how we review and develop talent. Our approach combines several advanced capabilities:
Talent Intelligence: AI analyzes skills gaps and development needs across our organization. This helps us make smart decisions about training and development investments.
Predictive Skills Analysis: AI now helps us forecast which skills will be most valuable for our organization’s future. Talent professionals should prepare for AI through reskilling and upskilling initiatives.
AI-driven skills assessment helps us create precise and effective workforce planning strategies. These tools excel at identifying both technical capabilities and soft skills, giving us a complete view of our talent landscape.
AI Governance and Compliance Language
AI governance language has become essential as we guide ourselves through compliance and ethics complexities. Organizations now focus more on creating clear frameworks that line up AI systems with legal requirements and ethical standards.
Regulatory compliance terms
Our work with AI compliance shows organizations must follow specific guidelines and legal requirements. The field grows faster in all sectors, and many of us still lack common ground on AI governance terms.
We work with several key compliance frameworks:
- AI Compliance Framework: Guidelines ensuring AI systems meet legal, ethical, and operational standards
- Conformity Assessment: Systematic evaluation process for high-risk AI systems
- Accountability Mechanisms: Systems ensuring AI actions can be traced to responsible entities
Latest data reveals 55.4% of organizations don’t have designated roles for responsible AI integration. This gap needs attention, especially since 27% of organizations call regulatory expertise a top concern.
Ethics and responsibility concepts
Ethical AI development that lines up with human values takes center stage now. Our all-encompassing approach to AI ethics covers several fundamental principles that guide responsible development:
Ethical Framework Components:
- Transparency: Clear communication about how AI algorithms operate
- Fairness: Prevention of discriminatory practices
- Accountability: Proactive standards management
Bias can creep into AI systems even with strong technology. Healthcare provides a striking example where a widely-used AI system showed major racial bias – identifying only 18% Black patients compared to 82% white patients for high-risk care, while actual distribution should have been 46% and 53% respectively.
Risk management vocabulary
Risk management strategies have evolved to tackle AI systems’ unique challenges. NIST’s AI Risk Management Framework helps us manage risks to individuals, organizations, and society better. Key areas demand our attention:
Risk Assessment Components:
- Impact Assessment: Evaluating potential ethical, legal, and societal implications
- AI Assurance: Including conformity assessments, audits, and certifications
- Monitoring Systems: Continuous oversight of AI operations
Reliable governance structures now include ethics boards and oversight committees. Experience teaches us that effective AI governance needs involvement from AI developers, users, policymakers, and ethicists.
Non-compliance carries heavy financial consequences. EU AI Act penalties range from EUR 7.5 million or 1.5% of worldwide annual turnover to EUR 35 million or 7% of worldwide annual turnover, depending on the violation.
Future-Ready Leadership AI Terms
Success in the AI era needs a new vocabulary that shapes how we stimulate innovation and handle change. Organizations preparing for an AI-driven future need more than just technical knowledge. They need a culture that welcomes continuous learning and adaptation.
Innovation and scaling terminology
AI integration needs a fresh approach to innovation. Recent data shows 44% of company leaders say their employees need new skills for the AI-driven business environment. This led us to create new frameworks to scale AI initiatives:
Innovation Metrics Table:
Component | Purpose | Impact |
---|---|---|
Value Creation | Measuring AI ROI | Business Growth |
Skill Development | Team Capability | Innovation Capacity |
Technology Integration | System Enhancement | Operational Efficiency |
Companies that successfully integrate AI gain exceptional competitive advantages. This needs more than new tools – it needs a fundamental change in our approach to leadership and innovation.
Digital transformation concepts
Our digital transformation experience taught us that AI deployment reshapes operational efficiency and decision-making. We focus on these key concepts:
- Adaptive Leadership: Moving from directive to facilitative approaches
- Digital Readiness: Assessing and building organizational capabilities
- Innovation Culture: Building environments that promote experimentation
- Strategic Alignment: Ensuring AI initiatives support business goals
About 84% of change practitioners now know AI moderately well. This suggests growing awareness of its strategic importance. AI isn’t just IT’s responsibility—it’s a leadership must-have.
Change management vocabulary
Our AI era change management focuses on three vital areas:
- Cultural Evolution Successful AI integration goes beyond technology understanding. It needs a culture that welcomes change and continuous learning. We run regular workshops, training sessions, and discussion groups about the latest AI tools and best practices.
- Leadership Development Leaders now enable rather than direct. Teams use AI for routine tasks while we plan strategy and build human relationships.
- Resistance Management We handle AI adoption challenges systematically. Our data reveals 60% of respondents expect major disruption from generative AI. We manage this transition by:
- Running regular AI literacy workshops
- Creating support systems for skill development
- Building communities of AI-driven leaders
AI integration into business operations changes how we envision and run businesses. Leadership in the AI era needs an open mindset that stays resilient during setbacks and commits to ethical stewardship.
Mutually beneficial alliances with academic institutions, tech companies, and industry organizations keep us current with latest developments. This collaborative approach gives our AI initiatives a well-rounded perspective.
Our experience proves that successful AI implementation balances investments with strategic planning for sustainable growth. We recruit experts in AI, machine learning, and data analysis. We also invest in scalable computing infrastructure to provide computational power for AI initiatives.
Conclusion
Business leadership today demands a solid understanding of AI terminology. AI affects every business operation from strategic planning and financial management to marketing and HR. The technology advances faster each day, and knowledge of these key terms enables informed decisions about AI implementation and investment.
The AI terminology experience shows that success requires more than technical knowledge. Leaders who learn these concepts can better assess AI solutions and communicate with technical teams. They can also guide their organizations through digital transformation more effectively. Data indicates that companies who embrace AI terminology and concepts achieve better outcomes in their AI initiatives.
New technologies will continue to shape AI terminology. The fundamental concepts discussed here are the foundations for business leaders. AI serves best as a tool to improve human capabilities rather than replace them. A clear understanding of these terms helps build effective strategies and leads to better AI adoption decisions.
FAQs
Q1. How can business leaders effectively integrate AI into their operations? Business leaders can integrate AI by focusing on specific business needs, taking an incremental approach, and viewing AI as an augmentation of human capabilities rather than a replacement. It’s important to foster a culture of continuous learning and adaptation while ensuring AI initiatives align with strategic goals.
Q2. What are some key AI terms every business leader should know? Essential AI terms for business leaders include machine learning, predictive analytics, natural language processing, deep learning, and neural networks. Understanding these concepts helps in making informed decisions about AI implementation and investment.
Q3. How is AI transforming marketing and customer experience? AI is revolutionizing marketing through advanced customer analytics, personalization technologies, and predictive modeling. It enables more precise targeting, real-time content adaptation, and hyper-personalized interactions, leading to improved customer experiences and higher conversion rates.
Q4. What are the main challenges in AI governance and compliance? Key challenges in AI governance include establishing clear ethical frameworks, ensuring regulatory compliance, managing bias in AI systems, and implementing effective risk assessment mechanisms. Organizations need to develop robust governance structures and involve various stakeholders to address these challenges.
Q5. How can leaders prepare their workforce for AI integration? Leaders can prepare their workforce by investing in AI literacy programs, creating support systems for skill development, and fostering a culture that embraces change. It’s crucial to focus on both technical skills and soft skills, while also addressing potential resistance through clear communication and change management strategies.