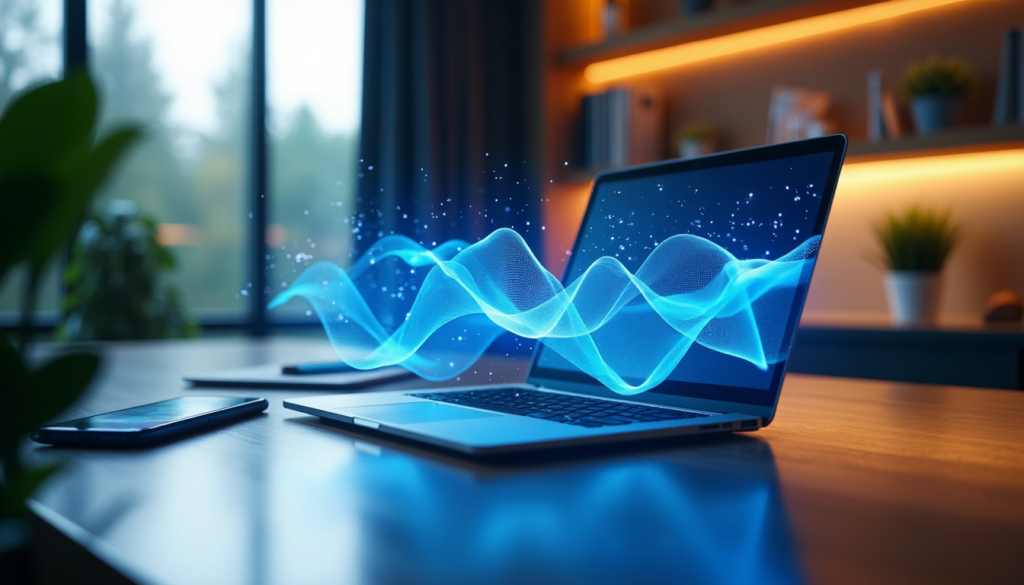
Introducing AI and the Role of Data
Let’s face it—AI is like the superhero of the tech world, swooping in to make our lives easier and more efficient. But even superheroes need their secret sauce, and for AI, that secret sauce is data. Think of data as the lifeblood that keeps AI from being just another buzzword. Without it, your smart assistant wouldn’t be so smart, your recommendations wouldn’t be so spot-on, and those chatbots would be as clueless as a fish out of water.
Imagine trying to teach a toddler to recognize a cat without ever showing them a picture of one. Pretty tough, right? That’s exactly what AI faces without data. Data provides the examples, the scenarios, and the context that AI needs to learn and grow. Just like we get better at things with practice, AI gets better the more data it processes.
Now, not all data is created equal. You wouldn’t fuel a sports car with vegetable oil, and you shouldn’t expect AI to perform miracles with subpar data. Quality matters. High-quality, diverse data sets are what make AI truly shine. They allow it to adapt, learn, and tackle a wide range of tasks, from diagnosing diseases to driving cars.
But it’s not just about having data; it’s about having the *right* data. The variety and volume of data feed the AI engine, helping it recognize patterns and make accurate predictions. The more it sees, the better it gets, much like how we humans learn from our experiences.
In the grand scheme of things, data is what separates a mediocre AI from a game-changing one. It’s the unsung hero, working behind the scenes to ensure that your AI applications can handle anything you throw at them. So, the next time you’re wowed by how accurately your music app recommends songs, remember—it’s all thanks to the treasure trove of data fueling those algorithms.
Data as the Lifeblood of AI
Data is to AI what water is to plants—absolutely essential. Just like plants soak up water to grow tall and strong, AI systems munch on data to get smarter and more efficient. Picture your AI as a brainy sponge, absorbing every drop of information to make sense of the world and solve problems faster than you can say “machine learning.”
Now, not all data is equal. Some data is like a gourmet meal, rich and varied, giving AI the nutrients it needs to tackle complex tasks. Other data, not so much—it’s more like fast food, quick and easy but not very nourishing. The best AI systems thrive on high-quality, diverse datasets that expose them to a broad spectrum of scenarios. This allows AI to adapt to new situations and handle everything from predicting stock market trends to diagnosing illnesses
.
Diversity in data is crucial. Imagine teaching a kid to recognize different dog breeds but only showing them pictures of Golden Retrievers. Sure, they’ll become an expert on Golden Retrievers, but show them a Poodle and they’ll be stumped. AI needs exposure to varied data to understand the nuances and complexities of real-world situations.
Volume also matters. Think of it as the difference between studying for an exam with a single page of notes versus a whole textbook. The more data AI has to train on, the more examples it can learn from, and the better it becomes at making accurate predictions. Large datasets act as a treasure chest of knowledge, enabling AI to learn from numerous instances and improve its decision-making abilities.
But let’s not forget, quality over quantity is key. Feeding AI with clean, well-organized, and relevant data is like giving a sports car high-octane fuel. It’s what enables AI to perform at its peak, making it a powerhouse capable of handling tasks that were once the stuff of science fiction.
In the end, data is the magic ingredient that transforms AI from a theoretical concept into a practical tool that can make life easier, more efficient, and even a little bit magical. So, the next time you marvel at how your favorite streaming service knows just what you want to watch, tip your hat to the incredible data working behind the scenes.
Obstacles in Gathering Data
Okay, let’s talk about the not-so-glamorous side of AI development—gathering data. If you think collecting seashells on a beach sounds tedious, imagine doing it blindfolded while juggling flaming torches. Yeah, it can be that tricky. One of the biggest headaches? Data privacy. With everyone more aware of how their personal information is handled, companies have to walk a tightrope. They need enough data to make their AI systems smart but must also ensure that your Aunt Linda’s cookie recipe doesn’t end up in some data leak scandal.
Then there’s the whole issue of data quality. Picture this: you’re making a gourmet meal and realize halfway through that half your ingredients are rotten. Not ideal, right? Similarly, feeding AI substandard data is like asking a gourmet chef to cook with expired ingredients. Poor-quality data leads to poor-quality results, and nobody wants an AI that’s as useful as a screen door on a submarine.
Data collection is also a logistical nightmare. Think about herding cats, except each cat represents a different piece of data from a different source. Some data comes from social media, some from sensors, and some from transaction logs. Pulling it all together into a cohesive dataset is like trying to fit mismatched puzzle pieces into a picture of a unicorn—it’s complicated
.
And let’s not forget the ethical implications. While it might be tempting to gather data from every possible source, doing so can lead to ethical quagmires. Is it really okay to scrape data from public forums without consent? What about using data from countries with different privacy laws? These questions keep data scientists up at night, sipping their umpteenth cup of coffee.
Oh, and did I mention the cost? Gathering data isn’t cheap. It’s not like you can just snap your fingers and have all the data you need magically appear. There are costs involved in acquiring, storing, and processing data. It’s like maintaining a sports car: the better the data, the higher the cost, but the performance is worth it.
So, as you can see, while data is the fuel that powers AI, collecting it is no walk in the park. It’s more like an epic quest, full of obstacles, challenges, and maybe even a few dragons to slay along the way.
Analyzing and Processing Data
Alright, now that we’ve braved the wilds of data collection, let’s dive into the next adventure: analyzing and processing that data. Think of raw data as a heap of unassembled Lego pieces—it’s all there, but without some serious sorting and building, you’ve just got a colorful mess. This is where sophisticated algorithms come in, acting like a master builder with a plan. They sift through the raw data, finding patterns, connections, and those sneaky outliers that don’t quite fit.
Imagine you’re hosting a dinner party, and you’ve got a pantry full of random ingredients. To whip up a gourmet meal, you need recipes and cooking skills. Similarly, AI algorithms take raw data and transform it into something useful. These algorithms are like chefs in a kitchen, combining ingredients in just the right way to make something deliciously insightful. They look for trends, relationships, and anomalies, turning what was once just noise into valuable information
.
But here’s the kicker: it’s not a one-size-fits-all kind of deal. Different AI tasks need different types of processing. For example, analyzing social media trends requires algorithms that can handle vast amounts of text data and understand context and sentiment. On the other hand, diagnosing medical conditions might require image recognition algorithms to interpret X-rays or MRIs.
Speed is also crucial. We’re living in the age of instant gratification, and nobody wants to wait around while their AI twiddles its virtual thumbs. Efficient algorithms process data quickly, enabling real-time decision-making. It’s like having a sous-chef who preps everything in record time, so you can get straight to the fun part—cooking up solutions.
And let’s not forget accuracy. Analyzing and processing data is pointless if the results aren’t reliable. That’s why these algorithms are designed to be precise, ensuring that the insights they generate are both meaningful and actionable. So, whether it’s predicting the next big stock market move or figuring out which Netflix show you’re going to binge next, the real magic happens during this stage, turning raw data into golden insights.
The Ongoing Demand for Data
If you thought keeping up with the latest fashion trends was exhausting, try keeping an AI model up-to-date! AI needs a steady stream of fresh data to stay sharp and relevant. It’s a bit like a never-ending buffet—always serving up something new for the AI to munch on. Picture this: today’s data might show a trend, but tomorrow’s could flip the script entirely. That’s why AI systems need a continuous flow of information to stay on their A-game.
This constant demand isn’t just about volume; it’s about freshness and relevance. Think of it as stocking your fridge—you wouldn’t keep last month’s leftovers when you can have fresh groceries, right? New data helps refine AI’s learning processes and adapt to changing circumstances. Whether it’s analyzing market trends, updating language models, or improving recommendation systems, ongoing data input is crucial for maintaining peak performance
.
Take the tech behind self-driving cars, for instance. These vehicles need real-time data to navigate roads safely. Traffic patterns, weather conditions, and roadblocks are constantly changing, and so should the data informing the AI. Without timely updates, you might end up with a car that’s great at driving last year’s routes but clueless about today’s detours.
The stakes are just as high in healthcare. AI systems analyzing medical data must be fed the latest research findings and patient information to make accurate diagnoses and treatment recommendations. Imagine relying on a doctor who hasn’t read a medical journal in years—not exactly reassuring, right?
And let’s not forget the world of finance. Stock market algorithms thrive on up-to-the-minute data to make split-second trading decisions. One outdated data point could mean the difference between a profitable trade and a financial fiasco. It’s like trying to win a race with a map from the 1800s—not gonna happen!
So, while it might seem like AI systems are insatiable data hogs, this ongoing demand is what makes them smart, adaptable, and, ultimately, incredibly useful. It’s a 24/7 job keeping them fed, but the results are worth it.
Practical Uses in the Real World
When it comes to real-world applications, AI is like that Swiss Army knife you didn’t know you needed. For starters, more than half of businesses are utilizing AI to streamline and improve operations by optimizing workflows and enhancing productivity. Imagine a world where your office coffee machine predicts your caffeine cravings before you even feel them—okay, maybe we’re not there yet, but you get the idea!
Take cybersecurity, for example. AI is becoming the digital guard dog for many organizations, combating fraud and protecting sensitive information. A significant portion of organizations employ AI to detect anomalies and identify potential threats by detecting anomalies and identifying potential threats. It’s like having a vigilant night watchman who never sleeps.
And let’s not forget customer service. Those chatbots on your favorite shopping sites? They’re powered by AI and get better with every customer interaction. Gone are the days of waiting on hold for hours; now, you can get quick, accurate responses almost instantly. It’s like having a super-efficient assistant at your beck and call
.
In healthcare, AI is making waves by assisting doctors in diagnosing diseases and recommending treatments. It’s as if medical professionals have a super-brainy sidekick helping them make life-saving decisions. The accuracy and speed with which AI can process medical data can be the difference between life and death.
Lastly, AI’s prowess in the finance world can’t be ignored. Stock market algorithms analyze real-time data to make rapid trading decisions. These algorithms are the financial wizards behind those big stock market moves, ensuring that investments are as strategic as a game of chess.
So, whether it’s keeping your data safe, improving your shopping experience, or even saving lives, AI’s practical applications are numerous and impactful. The ongoing influx of data ensures that these systems keep getting smarter, more reliable, and indispensable.